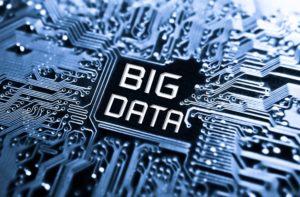
(wk1003mike/Shutterstock)
A humorous factor occurred on the best way to the AI promised land: Individuals realized they want information. Actually, they realized they want massive portions of all kinds of information, and that it could be higher if it was recent, trusted, and correct. In different phrases, folks realized they’ve a giant information drawback.
It might appear as if the world has moved past the “three Vs” of huge information–quantity, selection, and velocity (though with selection, veracity, and variability, you’re already as much as six). Now we have (fortunately) moved on from having to learn concerning the three (or six) Vs of information in each different article about trendy information administration.
To make certain, now we have made great progress on the technical entrance. Breakthroughs in {hardware} and software program–because of ultra-fast solid-state drives (SSDs), widespread 100GbE networks (and quicker), and most significantly of all, infinitely scalable cloud compute and storage–have helped us blow by way of outdated limitations that saved us from getting the place we needed.
Amazon S3 and related BLOB storage companies haven’t any theoretical restrict to the quantity of information they will retailer. And you’ll course of all that information to your coronary heart’s content material with the large assortment of cloud compute engines on Amazon EC2 and different companies. The one restrict there’s your pockets.
At the moment’s infrastructure software program can also be significantly better. One of the vital common huge information software program setups immediately is Apache Spark. The open supply framework, which rose to fame as a substitute for MapReduce in Hadoop clusters, has been deployed innumerable instances for a wide range of huge information duties, whether or not it’s constructing and working batch ETL pipelines, executing SQL queries, or processing huge streams of real-time information.
Databricks, the corporate began by Apache Spark’s creators, has been on the forefront of the lakehouse motion, which blends the scalability and adaptability of Hadoop-style information lakes with the accuracy and trustworthiness of conventional information warehouses.
Databricks senior vp of merchandise, Adam Conway, turned some heads with a LinkedIn article this week titled “Large Knowledge Is Again and Is Extra Essential Than AI.” Whereas huge information has handed the baton of hype off to AI, it’s huge information that individuals ought to be centered on, Conway mentioned.
“The truth is huge information is all over the place and it’s BIGGER than ever,” Conway writes. “Large information is flourishing inside enterprises and enabling them to innovate with AI and analytics in ways in which had been inconceivable just some years in the past.”
The scale of immediately’s information units definitely are huge. In the course of the early days of huge information, circa 2010, having 1 petabyte of information throughout your entire group was thought of huge. At the moment, there are corporations with 1PB of information in a single desk, Conway writes. The standard enterprise immediately has a knowledge property within the 10PB to 100PB vary, he says, and there are some corporations storing greater than 1 exabyte of information.
Databricks processes 9EBs of information per day on behalf of its shoppers. That definitely is a considerable amount of information, however when you think about all the corporations storing and processing information in cloud information lakes and on-prem Spark and Hadoop clusters, it’s only a drop within the bucket. The sheer quantity of information is rising yearly, as is the speed of information era.
However how did we get right here, and the place are we going? The rise of Internet 2.0 and social media kickstarted the preliminary huge information revolution. Big tech corporations like Fb, Twitter, Yahoo, LinkedIn, and others developed a variety of distributed frameworks (Hadoop, Hive, Storm, Presto, and so forth.) designed to allow customers to crunch large quantities of recent information varieties on business commonplace servers, whereas different frameworks, together with Spark and Flink, got here out of academia.
The digital exhaust flowing from on-line interactions (click on streams, logs) offered new methods of monetizing what folks see and do on screens. That spawned new approaches for coping with different huge information units, resembling IoT, telemetry, and genomic information, spurring ever extra product utilization and therefore extra information. These distributed frameworks had been open sourced to speed up their growth, and shortly sufficient, the massive information group was born.
Corporations do a wide range of issues with all this huge information. Knowledge scientists analyze it for patterns utilizing SQL analytics and classical machine studying algorithms, then prepare predictive fashions to show recent information into perception. Large information is used to create “gold” information units in information lakehouses, Conway says. And at last, they use huge information to construct information merchandise, and finally to coach AI fashions.
Because the world turns its consideration to generative AI, it’s tempting to suppose that the age of huge information is behind us, that we’ll bravely transfer on to tackling the following huge barrier in computing. Actually, the alternative is true. The rise of GenAI has proven enterprises that information administration within the period of huge information is each tough and vital.
“A lot of an important income producing or value saving AI workloads depend upon large information units,” Conway writes. “In lots of circumstances, there isn’t any AI with out huge information.”
The truth is that the businesses which have performed the arduous work of getting their information homes so as–i.e. those that have applied the methods and processes to have the ability to remodel massive quantities of uncooked information into helpful and trusted information units–have been those most readily capable of benefit from the brand new capabilities that GenAI have offered us.
That outdated mantra, “rubbish in, rubbish out,” has by no means been extra apropos. With out good information, the chances of constructing a very good AI mannequin are someplace between slim and none. To construct trusted AI fashions, one will need to have a practical information governance program in place that may guarantee the information’s lineage hasn’t been tampered with, that it’s secured from hackers and unauthorized entry, that non-public information is saved that approach, and that the information is correct.
As information grows in quantity, velocity, and all the opposite Vs, it turns into more durable and more durable to make sure good information administration and governance practices are in place. There are paths obtainable, as we cowl each day in these pages. However there aren’t any shortcuts or straightforward buttons, as many corporations are studying.
So whereas the way forward for AI is definitely vivid, the AI of the longer term will solely be nearly as good as the information that the AI is skilled on, or nearly as good as the information that’s gathered and despatched to the AI mannequin as a immediate. AI is ineffective with out good information. In the end, that will probably be huge information’s endearing legacy.
Associated Gadgets:
Informatica CEO: Good Knowledge Administration Not Elective for AI
Knowledge High quality Is A Mess, However GenAI Can Assist
Large Knowledge Is Nonetheless Exhausting. Right here’s Why