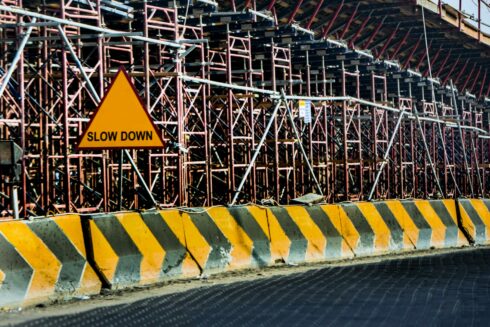
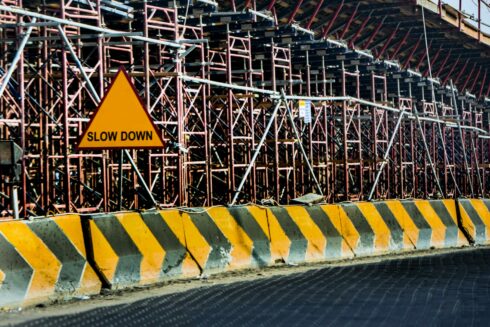
As synthetic intelligence advances at breakneck pace, utilizing it safely whereas additionally rising its workload is a essential concern. Conventional strategies of coaching protected AI have centered on filtering coaching knowledge or fine-tuning fashions post-training to mitigate dangers. Nevertheless, in late Might, Anthropic created a detailed map of the internal workings of its Claude 3 Sonnet mannequin, revealing how neuron-like options have an effect on its output. These interpretable options, which could be understood throughout languages and modalities like sound or pictures, are essential for bettering AI security. Options contained in the AI can spotlight, in actual time, how the mannequin is processing prompts and pictures. With this info, it’s potential to make sure that production-grade fashions keep away from bias and undesirable behaviors that would put security in danger.
Massive language fashions, resembling Claude 3 alongside its predecessor, Claude 2, and rival mannequin GPT-4, are revolutionizing how we work together with know-how. As all of those AI fashions achieve intelligence, security turns into the essential differentiator between them. Taking steps to extend interpretability units the stage to make AI actions and choices clear, de-risking the scaled-up use of AI for the enterprise.
Explainability Lays the Basis for Secure AI
Anthropic’s paper acts like an FMRI for the “Sonnet” AI mannequin, offering an unprecedented view into the intricate layers of language fashions. Neural networks are famously difficult. As Emerson as soon as mentioned, “If our brains have been so easy that we may perceive them, we’d not be capable to perceive them!”
Appreciable analysis has centered on understanding how self-taught studying techniques function, notably unsupervised or auto-encoder fashions that study from unlabelled knowledge with out human intervention. Higher understanding may result in extra environment friendly coaching strategies, saving time and power whereas enhancing precision, pace, and security.
Historic research on visible fashions, a number of the earliest and largest earlier than the arrival of language fashions, visually demonstrated how every subsequent layer within the mannequin provides complexity. Preliminary layers may establish easy edges, whereas deeper layers may discern corners and even full options like eyes.
By extending this understanding to language fashions, analysis exhibits how layers evolve from recognizing primary patterns to integrating complicated contexts. This creates AI that responds constantly to all kinds of associated inputs—an attribute often known as “invariance.” For instance, a chart exhibiting how a enterprise’ gross sales improve over time may set off the identical habits as a spreadsheet of numbers or an analysts’ remarks discussing the identical info. Thought unimaginable simply two years in the past, the impression of this “intelligence on faucet” for enterprise can’t be underestimated, as long as it’s dependable, truthful, and unbiased…in a phrase, protected.
Anthropic’s analysis lays the groundwork for integrating explainability from the outset. This proactive strategy will affect future analysis and growth in AI security.
The Promise of Opus! Demonstrating Scalability
Anthropic’s Opus is poised to scale these rules to a a lot bigger mannequin by proving the success of Sonnet’s interpretability, testing whether or not these options maintain at an excellent grander scale. Key questions embrace whether or not larger ranges in Opus are extra summary and complete, and if these options stay comprehensible to us or surpass our cognitive capabilities.
With evolutions in AI security and interpretability, opponents might be compelled to comply with go well with. This might usher in a brand new wave of analysis centered on creating clear and protected AI techniques throughout the trade.
This comes at an vital time. As LLMs proceed to advance in pace, context home windows, and reasoning, their potential functions in knowledge evaluation are increasing. The combination of fashions like Claude 3 and GPT-4 exemplifies the cutting-edge potentialities in fashionable knowledge analytics by simplifying complicated knowledge processing and paving the way in which for custom-made, extremely efficient enterprise intelligence options.
Whether or not you’re an information scientist, a part of an insights and analytics staff, or a Chief Expertise Officer, understanding these language fashions might be advantageous for unlocking their potential to reinforce enterprise operations throughout numerous sectors.
Steering for Explainable Fashions
A sensible strategy to attaining explainability is to have language fashions articulate their decision-making processes. Whereas this could result in rationalizations, sound logic will guarantee these explanations are sturdy and dependable. One strategy is to ask a mannequin to generate step-by-step guidelines for decision-making. This technique, particularly for moral choices, ensures transparency and accountability, filtering out unethical attributes whereas preserving requirements.
For non-language fashions, explainability could be achieved by figuring out “neighbors.” This entails asking the mannequin to supply examples from its coaching knowledge which can be just like its present determination, providing perception into the mannequin’s thought course of. An identical idea often known as “assist vectors” asks the mannequin to decide on examples that it believes separate one of the best choices for a choice that it has to make.
Within the context of unsupervised studying fashions, understanding these “neighbors” helps make clear the mannequin’s decision-making path, probably decreasing coaching time and energy necessities whereas enhancing precision and security.
The Way forward for AI Security and Massive Language Fashions
Anthropic’s current strategy to protected AI not solely paves the way in which for safer AI techniques but in addition units a brand new trade customary that prioritizes transparency and accountability from the bottom up.
As for the way forward for enterprise analytics, giant language fashions ought to start transferring in the direction of specialization of duties and clusters of cooperating AIs. Think about deploying a cheap and swift mannequin to course of uncooked knowledge, adopted by a extra subtle mannequin that synthesizes these outputs. A bigger context mannequin then evaluates the consistency of those outcomes in opposition to in depth historic knowledge, guaranteeing relevance and accuracy. Lastly, a specialised mannequin devoted to reality verification and hallucination detection scrutinizes these outputs earlier than publication. This layered technique, often known as a “graph” strategy, would cut back prices whereas enhancing output high quality and reliability, with every mannequin within the cluster optimized for a selected activity, thus offering clearer insights into the AI’s decision-making processes.
Incorporating this right into a broader framework, language fashions develop into an integral part of infrastructure—akin to storage, databases, and compute sources—tailor-made to serve various trade wants. As soon as security is a core function, the main focus could be on leveraging the distinctive capabilities of those fashions to reinforce enterprise functions that may present end-users with highly effective productiveness suites.