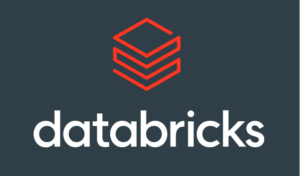
Final yr in December, Databricks, a number one supplier of knowledge intelligence and AI options, introduced a brand new suite of instruments to get GenAI functions to manufacturing utilizing Retrieval Augmented Era (RAG). Since then, we have now witnessed a speedy rise in RAG functions as enterprises are investing closely in constructing GenAI functions.
Conventional language fashions include a novel set of challenges, together with their tendency to “hallucinate”, lack of entry to vital info past their coaching datasets, and the lack to include real-time knowledge. RAG steps in as an answer to a few of these points by combining its retrieval capabilities with its means to generate pure language.
To assist make it simple for enterprises to construct high-quality RAG functions, Databricks has introduced a number of updates to its platform, together with the overall availability of Vector Seek for fast and correct retrieval of related info.
Mannequin Serving, Databricks’ setting for creating and managing AI and ML fashions, has additionally been up to date to supply a extra intuitive UI, assist for added LLMs, efficiency enhancements, and higher governance and auditability.
Databricks is named a knowledge lakehouse pioneer, seamlessly integrates the structured knowledge administration functionalities of a knowledge warehouse with the unstructured knowledge administration capabilities of a knowledge lake. Just lately, the corporate has been specializing in strategic enlargement, with a brand new partnership with Tableau to allow extra seamless and safe knowledge interplay, and expanded collaboration with NVIDIA to speed up knowledge and AI workloads.
“Builders spend an inordinate quantity of effort and time to make sure that the output of AI functions is correct, protected, and ruled earlier than making it accessible to their clients and infrequently cite accuracy and high quality as the most important blockers to unlocking the worth of those thrilling new applied sciences.” shared Databricks in a weblog submit.
In response to Databricks, LLM builders have historically targeted on offering the very best high quality baseline reasoning and information capabilities, nonetheless, latest analysis reveals that that is certainly one of many determinants of the general high quality of the AI functions. Incorporating a broader enterprise context, establishing correct governance and entry controls, and having a deeper understanding of knowledge are among the different elements which might be vital to the standard of the AI utility.
The brand new updates to the Databricks platform deal with a few of these issues by including extra enterprise context and steerage to ascertain a higher understanding of knowledge.
As well as, the updates supply a extra complete strategy that covers a number of elements by means of the GenAI course of, together with knowledge preparation, knowledge retrieval, knowledge coaching on enterprise knowledge, immediate engineering, and post-processing pipelines.
The addition of vector databases to the Databricks platform will allow coaching fashions to precisely perceive the distinctive traits of a person group to enhance retrieval velocity, response high quality, and accuracy.
As we navigate by means of the ever-increasing complexities of AI and chatbots, RAG stands out as a beacon of innovation. With its means to mix the huge information bases with the precision of retrieve-based info, RAG is poised to remodel our interactions with AI. We will anticipate extra enterprises to proceed embracing RAG to assist them unlock new potentialities of their technological journey.
Associated Gadgets
Taking GenAI from Good to Nice: Retrieval-Augmented Era and Actual-Time Information
Galileo Introduces RAG & Agent Analytics Resolution for Higher, Sooner AI Improvement
Harnessing Hybrid Intelligence: Balancing AI Fashions and Human Experience for Optimum Efficiency