Introduction
Giant language fashions (LLMs) have dramatically reshaped computational arithmetic. These superior AI programs, designed to course of and mimic human-like textual content, are actually pushing boundaries in mathematical fields. Their potential to know and manipulate advanced ideas has made them invaluable in analysis and improvement. Amongst these improvements stands Paramanu-Ganita, a creation of Gyan AI Analysis. This mannequin, although solely 208 million parameters sturdy, outshines lots of its bigger counterparts. It’s particularly constructed to excel in mathematical reasoning, demonstrating that smaller fashions can certainly carry out exceptionally nicely in specialised domains. On this article, we’ll discover the event and capabilities of the Paramanu-Ganita AI mannequin.
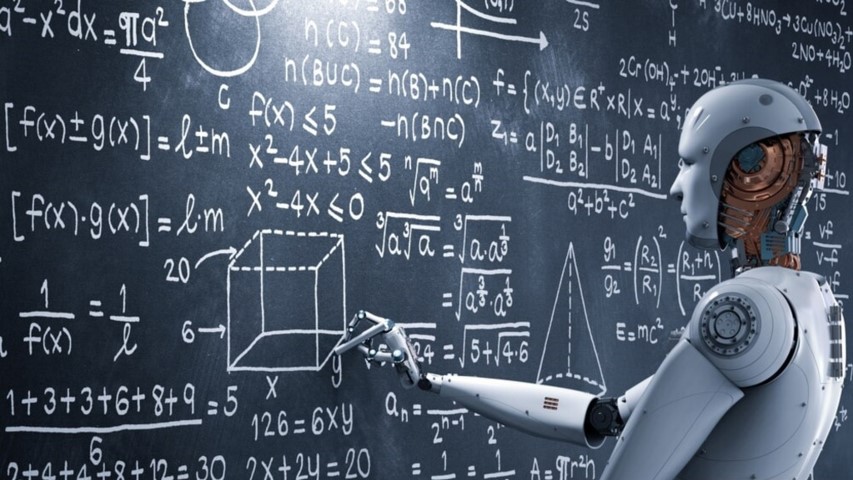
The Rise of Smaller Scale Fashions
Whereas large-scale LLMs have spearheaded quite a few AI breakthroughs, they arrive with important challenges. Their huge dimension calls for in depth computational energy and power, making them costly and fewer accessible. This has prompted a seek for extra viable alternate options.
Smaller, domain-specific fashions like Paramanu-Ganita show advantageous. By specializing in particular areas, reminiscent of arithmetic, these fashions obtain greater effectivity and effectiveness. Paramanu-Ganita, as an example, requires fewer assets and but operates sooner than bigger fashions. This makes it perfect for environments with restricted assets. Its specialization in arithmetic permits for refined efficiency, usually surpassing generalist fashions in associated duties.
This shift in direction of smaller, specialised fashions is prone to affect the longer term route of AI, notably in technical and scientific fields the place depth of information is essential.
Improvement of Paramanu-Ganita
Paramanu-Ganita was developed with a transparent focus: to create a robust, but smaller-scale language mannequin that excels in mathematical reasoning. This method counters the development of constructing ever-larger fashions. It as an alternative focuses on optimizing for particular domains to attain excessive efficiency with much less computational demand.
Coaching and Improvement Course of
The coaching of Paramanu-Ganita concerned a curated mathematical corpus, chosen to boost its problem-solving capabilities throughout the mathematical area. It was developed utilizing an Auto-Regressive (AR) decoder and educated from scratch. Impressively, it managed to achieve its targets with simply 146 hours of coaching on an Nvidia A100 GPU. That is only a fraction of the time bigger fashions require.
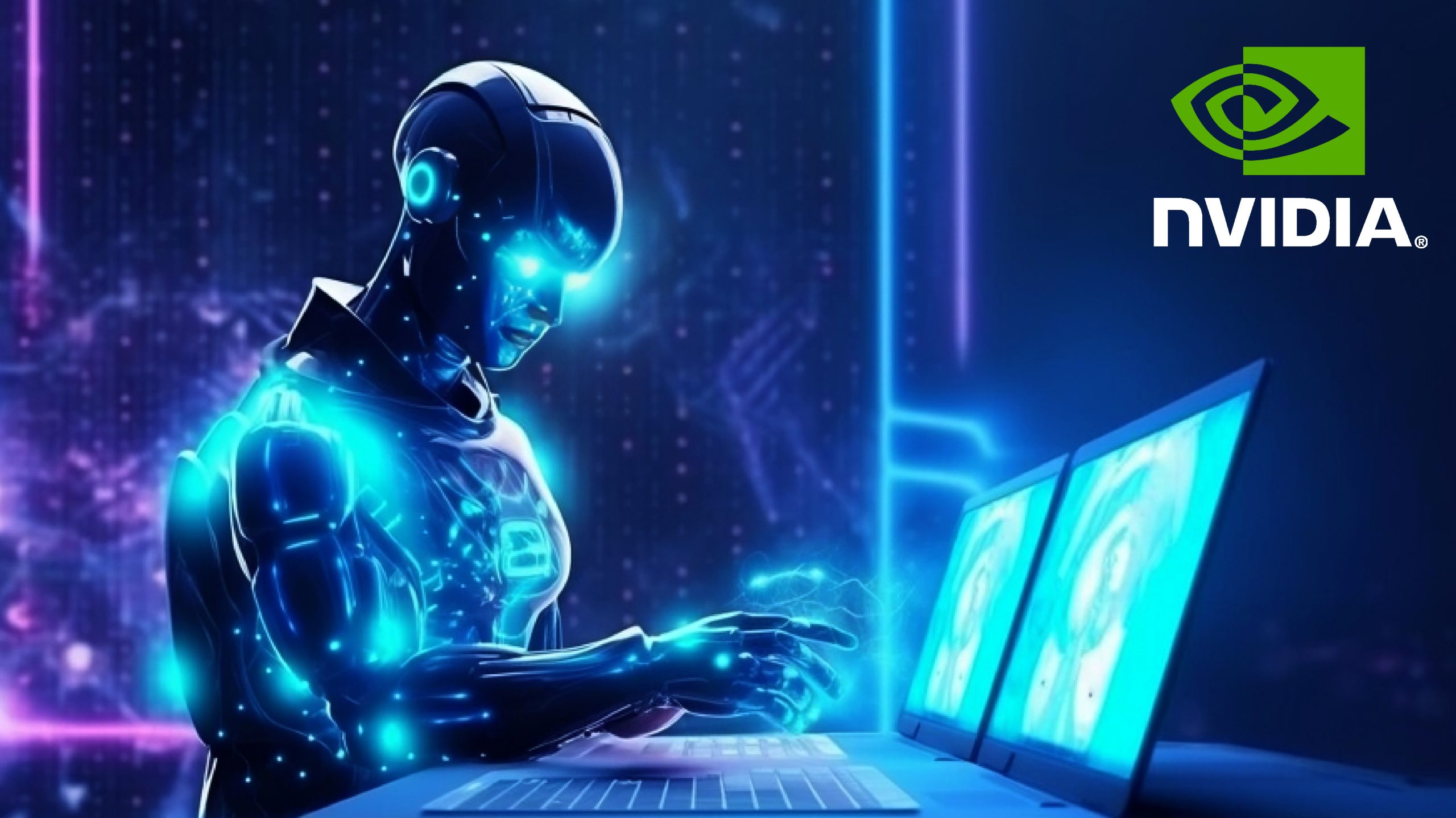
Distinctive Options and Technical Specs
Paramanu-Ganita stands out with its 208 million parameters, a considerably smaller quantity in comparison with the billions usually present in giant LLMs. This mannequin helps a big context dimension of 4096, permitting it to deal with advanced mathematical computations successfully. Regardless of its compact dimension, it maintains excessive effectivity and pace, able to operating on lower-spec {hardware} with out efficiency loss.
Efficiency Evaluation
Paramanu-Ganita’s design vastly enhances its potential to carry out advanced mathematical reasoning. Its success in particular benchmarks like GSM8k highlights its potential to deal with advanced mathematical issues effectively, setting a brand new commonplace for the way language fashions can contribute to computational arithmetic.
Comparability with Different LLMs like LLaMa, Falcon, and PaLM
Paramanu-Ganita has been immediately in contrast with bigger LLMs reminiscent of LLaMa, Falcon, and PaLM. It exhibits superior efficiency, notably in mathematical benchmarks, the place it outperforms these fashions by important margins. For instance, regardless of its smaller dimension, it outperforms Falcon 7B by 32.6% factors and PaLM 8B by 35.3% factors in mathematical reasoning.
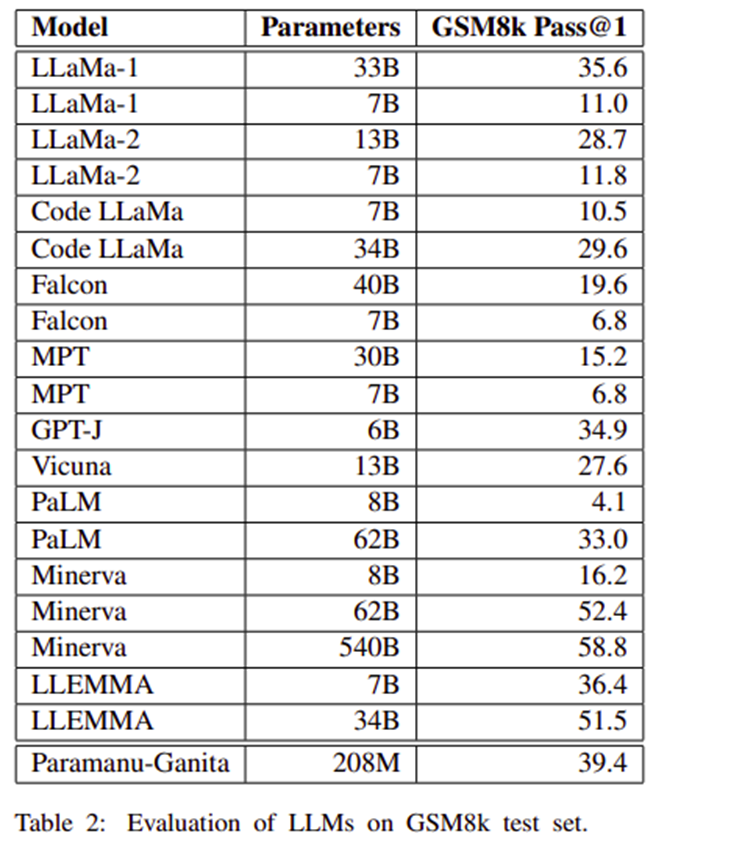
Detailed Efficiency Metrics on GSM8k Benchmarks
On the GSM8k benchmark, which evaluates the mathematical reasoning capabilities of language fashions, Paramanu-Ganita achieved outstanding outcomes. It scored greater than many bigger fashions, demonstrating a Cross@1 accuracy that surpasses LLaMa-1 7B and Falcon 7B by over 28% and 32% factors, respectively. This spectacular efficiency underlines its effectivity and specialised functionality in dealing with mathematical duties, confirming the success of its targeted and environment friendly design philosophy.
Implications and Improvements
One of many key improvements of Paramanu-Ganita is its cost-effectiveness. The mannequin requires considerably much less computational energy and coaching time in comparison with bigger fashions, making it extra accessible and simpler to deploy in numerous settings. This effectivity doesn’t compromise its efficiency, making it a sensible selection for a lot of organizations.
The traits of Paramanu-Ganita make it well-suited for academic functions, the place it could possibly help in instructing advanced mathematical ideas. In skilled environments, its capabilities will be leveraged for analysis in theoretical arithmetic, engineering, economics, and information science, offering high-level computational help.
Future Instructions
The event group behind Paramanu-Ganita is actively engaged on an intensive examine to coach a number of pre-trained mathematical language fashions from scratch. They goal to research whether or not numerous mixtures of assets—reminiscent of mathematical books, web-crawled content material, ArXiv math papers, and supply code from related programming languages—improve the reasoning capabilities of those fashions.
Moreover, the group plans to include mathematical question-and-answer pairs from in style boards like StackExchange and Reddit into the coaching course of. This effort is designed to evaluate the complete potential of those fashions and their potential to excel on the GSM8K benchmark, a preferred math benchmark.
By exploring these various datasets and mannequin sizes, the group hopes to additional enhance the reasoning potential of Paramanu-Ganita and probably surpass the efficiency of state-of-the-art LLMs, regardless of Paramanu-Ganita’s comparatively smaller dimension of 208 million parameters.
Paramanu-Ganita’s success opens the door to broader impacts in AI, notably in how smaller, specialised fashions might be designed for different domains. Its achievements encourage additional exploration into how such fashions will be utilized in computational arithmetic. Ongoing analysis on this area exhibits potential in dealing with algorithmic complexity, optimization issues, and past. Comparable fashions thus maintain the ability to reshape the panorama of AI-driven analysis and utility.
Conclusion
Paramanu-Ganita marks a major step ahead in AI-driven mathematical problem-solving. This mannequin challenges the necessity for bigger language fashions by proving that smaller, domain-specific options will be extremely efficient. With excellent efficiency on benchmarks like GSM8k and a design that emphasizes cost-efficiency and lowered useful resource wants, Paramanu-Ganita exemplifies the potential of specialised fashions to revolutionize technical fields. Because it evolves, it guarantees to broaden the influence of AI, introducing extra accessible and impactful computational instruments throughout numerous sectors, and setting new requirements for AI functions in computational arithmetic and past.