Discover India’s surge in semiconductor imports, the urgent want for self-reliance, and the strategic pillars reshaping the nation’s technological future.
Synthetic intelligence (AI) refers back to the skill of a pc system to duplicate human cognitive talents, together with studying and problem-solving expertise. Though AI and machine studying (ML) are intricately associated, they’re distinct ideas. Machine studying is considered a subset of AI, indicating that it’s a specialised space throughout the broader area of synthetic intelligence. The connection between synthetic intelligence and machine studying offers vital benefits for companies throughout various sectors, with consistently evolving alternatives and potential functions.
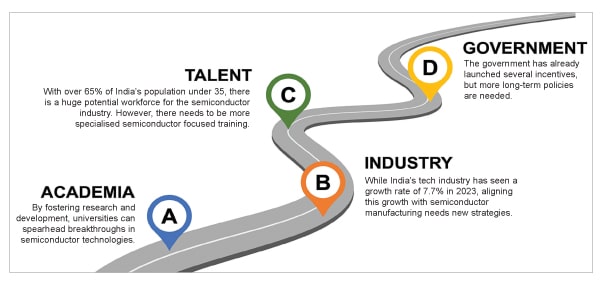
The mixing of AI and ML applied sciences has revolutionised varied industries, and materials manufacturing isn’t any exception. This text explores the numerous function AI and ML play in optimising and enhancing the whole materials manufacturing course of, from materials design and synthesis to high quality management and predictive upkeep. We delve into varied functions, challenges, and future prospects, showcasing how these applied sciences are contributing to the evolution of the fabric manufacturing sector.
Materials manufacturing has been a cornerstone of human civilisation, driving technological developments and financial development. Historically, materials improvement and manufacturing processes relied on empirical information, experimentation, and trial-and-error strategies. Nonetheless, with the appearance of AI and ML, the business has witnessed a transformative shift. These applied sciences supply highly effective instruments for optimising materials properties, enhancing manufacturing effectivity, and making certain product high quality.
Supplies design and synthesis
AI has revolutionised the supplies design and synthesis part by accelerating the introduction and predicting the interplay of novel supplies with desired properties. Researchers can leverage AI algorithms to foretell materials properties primarily based on chemical composition, crystal construction, structural interactions, and different bodily and chemical elements. ML fashions skilled on huge materials databases can generate precious insights, resulting in the creation of recent formulations tailor-made for particular functions.
Supplies property prediction
AI-driven supplies property prediction fashions can considerably cut back the time and price required for materials improvement. These fashions utilise knowledge from varied sources, equivalent to quantum calculations, experimental knowledge, and simulations, to foretell materials properties like mechanical energy, electrical and thermal conductivity, and thermal stability, and so forth. Researchers can use these predictions to establish promising candidates for additional examine, expediting the supplies formulation and synthesis course of. This could considerably cut back the time to reach on the proper materials makes use of and keep away from the trial and error technique within the laboratory.
Supplies informatics
Supplies informatics combines AI and ML strategies to extract precious data from huge datasets. By analysing knowledge associated to supplies’ synthesis strategies, properties, and efficiency, researchers can uncover hidden correlations and patterns. This information helps in optimising materials manufacturing processes, making certain higher efficiency and useful resource utilisation.