The unprecedented rise of synthetic intelligence (AI) has introduced transformative potentialities throughout the board, from industries and economies to societies at massive. Nevertheless, this technological leap additionally introduces a set of potential challenges. In its latest public assembly, the Nationwide AI Advisory Committee (NAIAC)1, which gives suggestions across the U.S. AI competitiveness, the science round AI, and the AI workforce to the President and the Nationwide AI Initiative Workplace, has voted on a advice on ‘Generative AI Away from the Frontier.’2
This advice goals to stipulate the dangers and proposed suggestions for methods to assess and handle off-frontier AI fashions – sometimes referring to open supply fashions. In abstract, the advice from the NAIAC gives a roadmap for responsibly navigating the complexities of generative AI. This weblog submit goals to make clear this advice and delineate how DataRobot clients can proactively leverage the platform to align their AI adaption with this advice.
Frontier vs Off-Frontier Fashions
Within the advice, the excellence between frontier and off-frontier fashions of generative AI is predicated on their accessibility and degree of development. Frontier fashions symbolize the newest and most superior developments in AI know-how. These are advanced, high-capability programs sometimes developed and accessed by main tech firms, analysis establishments, or specialised AI labs (reminiscent of present state-of-the-art fashions like GPT-4 and Google Gemini). As a consequence of their complexity and cutting-edge nature, frontier fashions sometimes have constrained entry – they aren’t broadly accessible or accessible to most people.
Alternatively, off-frontier fashions sometimes have unconstrained entry – they’re extra broadly accessible and accessible AI programs, usually accessible as open supply. They won’t obtain probably the most superior AI capabilities however are important because of their broader utilization. These fashions embrace each proprietary programs and open supply AI programs and are utilized by a wider vary of stakeholders, together with smaller firms, particular person builders, and academic establishments.
This distinction is necessary for understanding the completely different ranges of dangers, governance wants, and regulatory approaches required for varied AI programs. Whereas frontier fashions may have specialised oversight because of their superior nature, off-frontier fashions pose a distinct set of challenges and dangers due to their widespread use and accessibility.
What the NAIAC Advice Covers
The advice on ‘Generative AI Away from the Frontier,’ issued by NAIAC in October 2023, focuses on the governance and danger evaluation of generative AI programs. The doc gives two key suggestions for the evaluation of dangers related to generative AI programs:
For Proprietary Off-Frontier Fashions: It advises the Biden-Harris administration to encourage firms to increase voluntary commitments3 to incorporate risk-based assessments of off-frontier generative AI programs. This contains unbiased testing, danger identification, and data sharing about potential dangers. This advice is especially geared toward emphasizing the significance of understanding and sharing the knowledge on dangers related to off-frontier fashions.
For Open Supply Off-Frontier Fashions: For generative AI programs with unconstrained entry, reminiscent of open-source programs, the Nationwide Institute of Requirements and Know-how (NIST) is charged to collaborate with a various vary of stakeholders to outline applicable frameworks to mitigate AI dangers. This group contains academia, civil society, advocacy organizations, and the trade (the place authorized and technical feasibility permits). The aim is to develop testing and evaluation environments, measurement programs, and instruments for testing these AI programs. This collaboration goals to determine applicable methodologies for figuring out crucial potential dangers related to these extra overtly accessible programs.
NAIAC underlines the necessity to perceive the dangers posed by broadly accessible, off-frontier generative AI programs, which embrace each proprietary and open-source programs. These dangers vary from the acquisition of dangerous info to privateness breaches and the era of dangerous content material. The advice acknowledges the distinctive challenges in assessing dangers in open-source AI programs as a result of lack of a set goal for evaluation and limitations on who can check and consider the system.
Furthermore, it highlights that investigations into these dangers require a multi-disciplinary strategy, incorporating insights from social sciences, behavioral sciences, and ethics, to assist selections about regulation or governance. Whereas recognizing the challenges, the doc additionally notes the advantages of open-source programs in democratizing entry, spurring innovation, and enhancing inventive expression.
For proprietary AI programs, the advice factors out that whereas firms could perceive the dangers, this info is usually not shared with exterior stakeholders, together with policymakers. This requires extra transparency within the area.
Regulation of Generative AI Fashions
Not too long ago, dialogue on the catastrophic dangers of AI has dominated the conversations on AI danger, particularly almost about generative AI. This has led to calls to manage AI in an try to advertise accountable improvement and deployment of AI instruments. It’s value exploring the regulatory possibility almost about generative AI. There are two primary areas the place coverage makers can regulate AI: regulation at mannequin degree and regulation at use case degree.
In predictive AI, typically, the 2 ranges considerably overlap as slim AI is constructed for a selected use case and can’t be generalized to many different use circumstances. For instance, a mannequin that was developed to establish sufferers with excessive chance of readmission, can solely be used for this explicit use case and would require enter info much like what it was educated on. Nevertheless, a single massive language mannequin (LLM), a type of generative AI fashions, can be utilized in a number of methods to summarize affected person charts, generate potential therapy plans, and enhance the communication between the physicians and sufferers.
As highlighted within the examples above, not like predictive AI, the identical LLM can be utilized in a wide range of use circumstances. This distinction is especially necessary when contemplating AI regulation.
Penalizing AI fashions on the improvement degree, particularly for generative AI fashions, may hinder innovation and restrict the useful capabilities of the know-how. Nonetheless, it’s paramount that the builders of generative AI fashions, each frontier and off-frontier, adhere to accountable AI improvement tips.
As an alternative, the main focus ought to be on the harms of such know-how on the use case degree, particularly at governing the use extra successfully. DataRobot can simplify governance by offering capabilities that allow customers to judge their AI use circumstances for dangers related to bias and discrimination, toxicity and hurt, efficiency, and price. These options and instruments might help organizations be certain that AI programs are used responsibly and aligned with their present danger administration processes with out stifling innovation.
Governance and Dangers of Open vs Closed Supply Fashions
One other space that was talked about within the advice and later included within the just lately signed govt order signed by President Biden4, is lack of transparency within the mannequin improvement course of. Within the closed-source programs, the creating group could examine and consider the dangers related to the developed generative AI fashions. Nevertheless, info on potential dangers, findings round consequence of pink teaming, and evaluations performed internally has not typically been shared publicly.
Alternatively, open-source fashions are inherently extra clear because of their overtly accessible design, facilitating the simpler identification and correction of potential issues pre-deployment. However in depth analysis on potential dangers and analysis of those fashions has not been carried out.
The distinct and differing traits of those programs indicate that the governance approaches for open-source fashions ought to differ from these utilized to closed-source fashions.
Keep away from Reinventing Belief Throughout Organizations
Given the challenges of adapting AI, there’s a transparent want for standardizing the governance course of in AI to stop each group from having to reinvent these measures. Varied organizations together with DataRobot have provide you with their framework for Reliable AI5. The federal government might help lead the collaborative effort between the non-public sector, academia, and civil society to develop standardized approaches to deal with the issues and supply sturdy analysis processes to make sure improvement and deployment of reliable AI programs. The latest govt order on the protected, safe, and reliable improvement and use of AI directs NIST to steer this joint collaborative effort to develop tips and analysis measures to know and check generative AI fashions. The White Home AI Invoice of Rights and the NIST AI Threat Administration Framework (RMF) can function foundational rules and frameworks for accountable improvement and deployment of AI. Capabilities of the DataRobot AI Platform, aligned with the NIST AI RMF, can help organizations in adopting standardized belief and governance practices. Organizations can leverage these DataRobot instruments for extra environment friendly and standardized compliance and danger administration for generative and predictive AI.
1 Nationwide AI Advisory Committee – AI.gov
2 RECOMMENDATIONS: Generative AI Away from the Frontier
4 https://www.datarobot.com/trusted-ai-101/
Concerning the writer
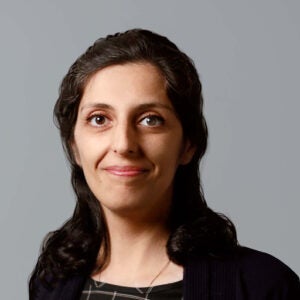
Haniyeh is a International AI Ethicist on the DataRobot Trusted AI crew and a member of the Nationwide AI Advisory Committee (NAIAC). Her analysis focuses on bias, privateness, robustness and stability, and ethics in AI and Machine Studying. She has a demonstrated historical past of implementing ML and AI in a wide range of industries and initiated the incorporation of bias and equity function into DataRobot product. She is a thought chief within the space of AI bias and moral AI. Haniyeh holds a PhD in Astronomy and Astrophysics from the Rheinische Friedrich-Wilhelms-Universität Bonn.
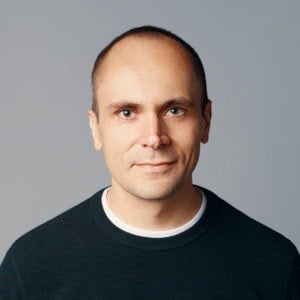
Michael Schmidt serves as Chief Know-how Officer of DataRobot, the place he’s chargeable for pioneering the following frontier of the corporate’s cutting-edge know-how. Schmidt joined DataRobot in 2017 following the corporate’s acquisition of Nutonian, a machine studying firm he based and led, and has been instrumental to profitable product launches, together with Automated Time Sequence. Schmidt earned his PhD from Cornell College, the place his analysis targeted on automated machine studying, synthetic intelligence, and utilized math. He lives in Washington, DC.