Introduction
Analysis of fashions and medical checks is critical in each knowledge science and medication. Nonetheless, these two domains use completely different metrics, which is complicated. Whereas knowledge scientists use precision and recall, medics use specificity and sensitivity. Relating to the connection between these metrics, they differ. This, due to this fact, requires comprehension of their disparities and functions to guage fashions precisely and have an efficient change between knowledge scientists and medical professionals.
Overview
- The weblog contrasts knowledge science metrics (precision, recall) with medical metrics (specificity, sensitivity) for mannequin analysis.
- Precision measures the accuracy of constructive predictions, whereas recall (sensitivity) assesses the detection of all precise positives.
- Specificity evaluates the accuracy of unfavourable predictions, which is essential for figuring out true negatives in medical checks.
- Sensible examples illustrate the implications of various metric combos in medical screenings and illness detection.
- Balancing precision and recall utilizing the F1 rating is beneficial for complete mannequin efficiency analysis.
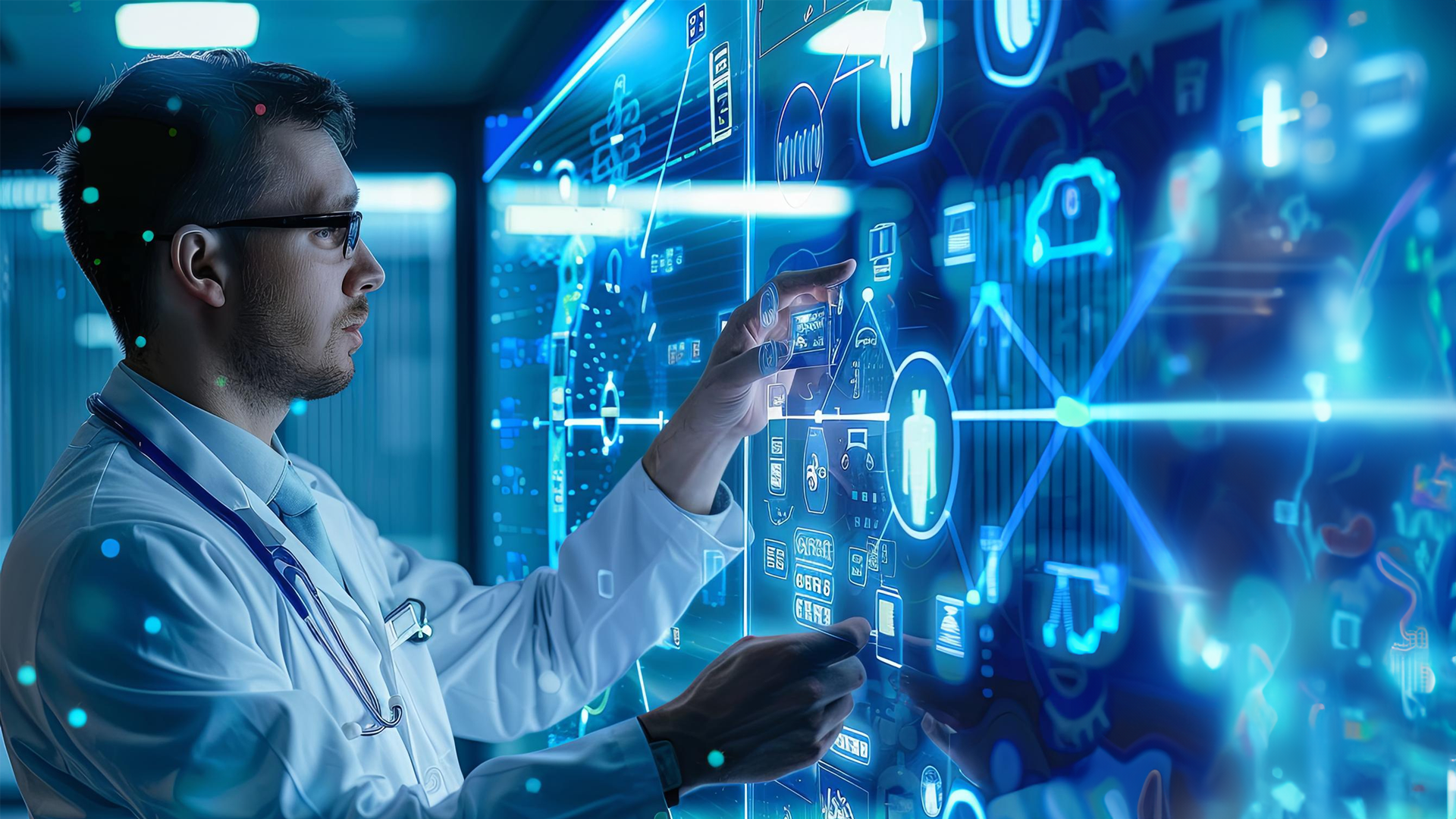
Knowledge Science Metrics
Precision is the ratio or fraction of true positives out of all constructive examples predicted by a mannequin. It solutions this query: “Out of all of the examples predicted as constructive, what number of are constructive?”
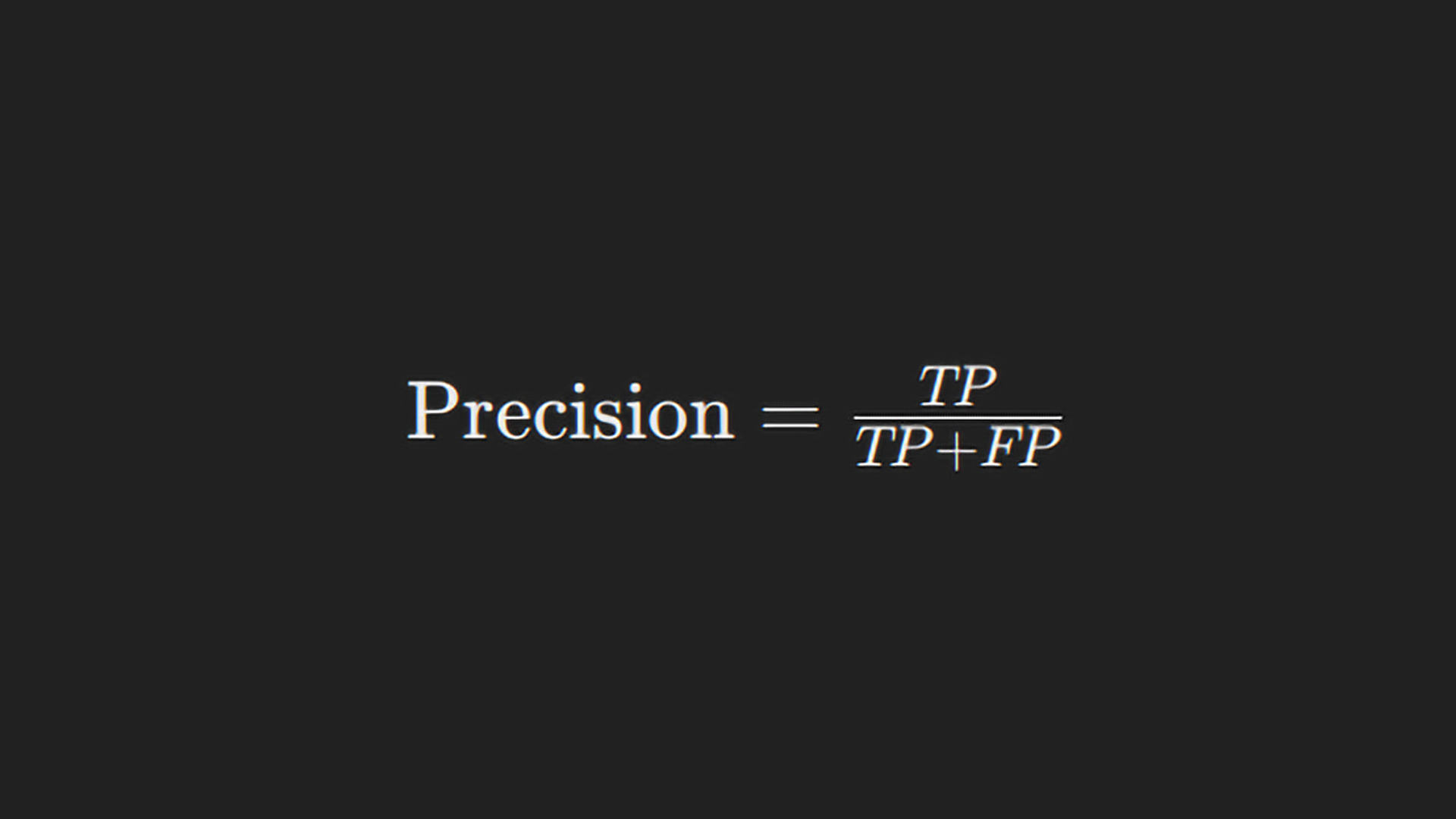
The whole variety of circumstances that had been positively categorised based mostly on their precise existence as HIV/AIDS might be measured utilizing precision.
Precision tells us how lots of the positively categorised cases had been constructive. As an illustration, in a spam detection system, precision calculates the proportion of emails marked as spam which are spam.
Recall, additionally known as sensitivity within the subject, measures the fraction of all true positives divided by whole precise constructive circumstances. It addresses the query: “Of all constructive circumstances, what number of had been accurately predicted as positives?”
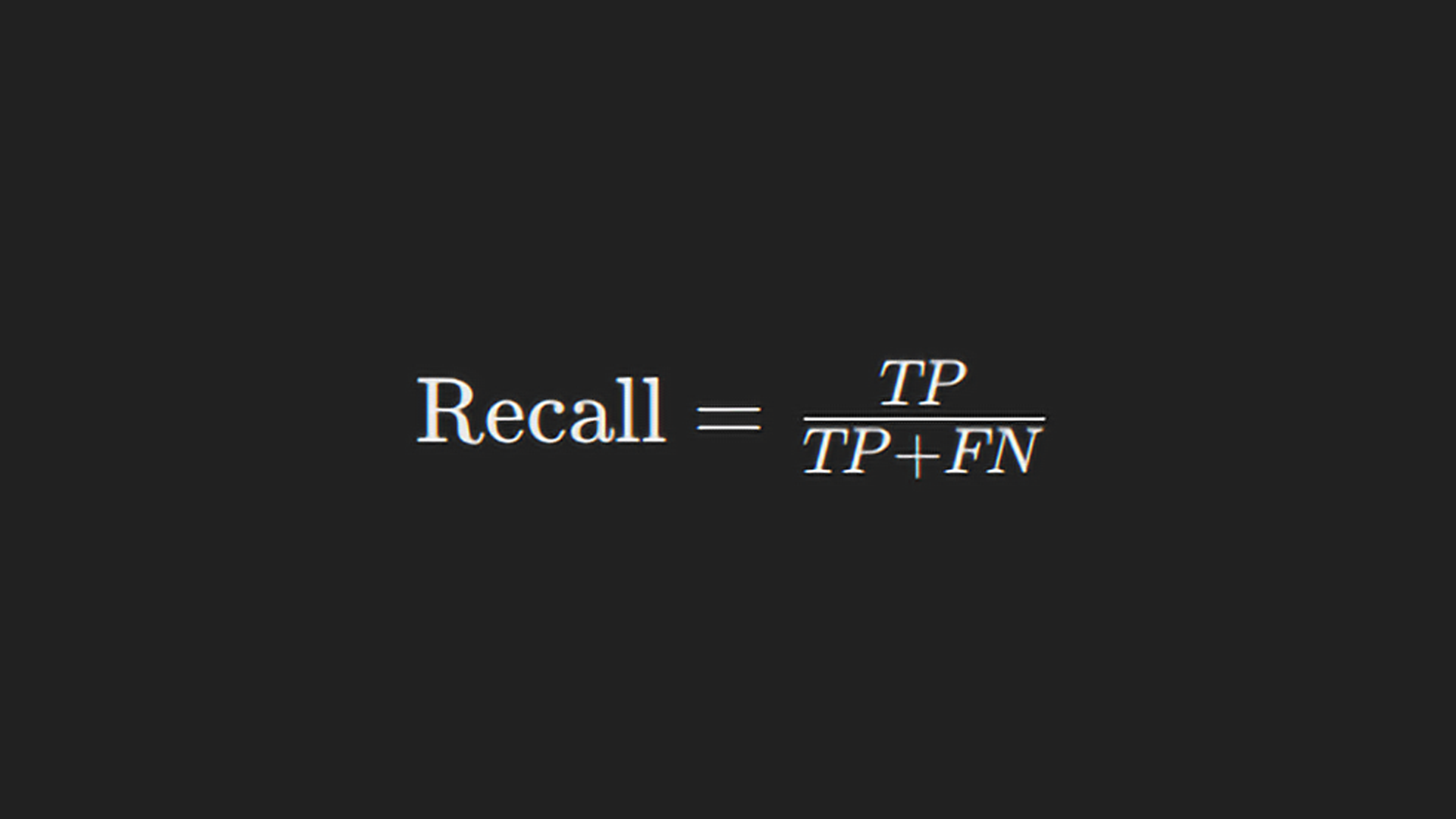
Recall refers back to the mannequin’s potential to seek out all related cases. For instance, within the case of a medical check for a illness, recall tells us what number of precise constructive circumstances (sufferers with the illness) had been accurately recognized by the check.
Additionally learn: Machine Studying & AI for Healthcare in 2024
Medical Metrics
Specificity calculates the ratio of true negatives predicted and people which are unfavourable. It seeks to reply the query, ” What number of unfavourable predictions for individuals who don’t have a situation are appropriate?”
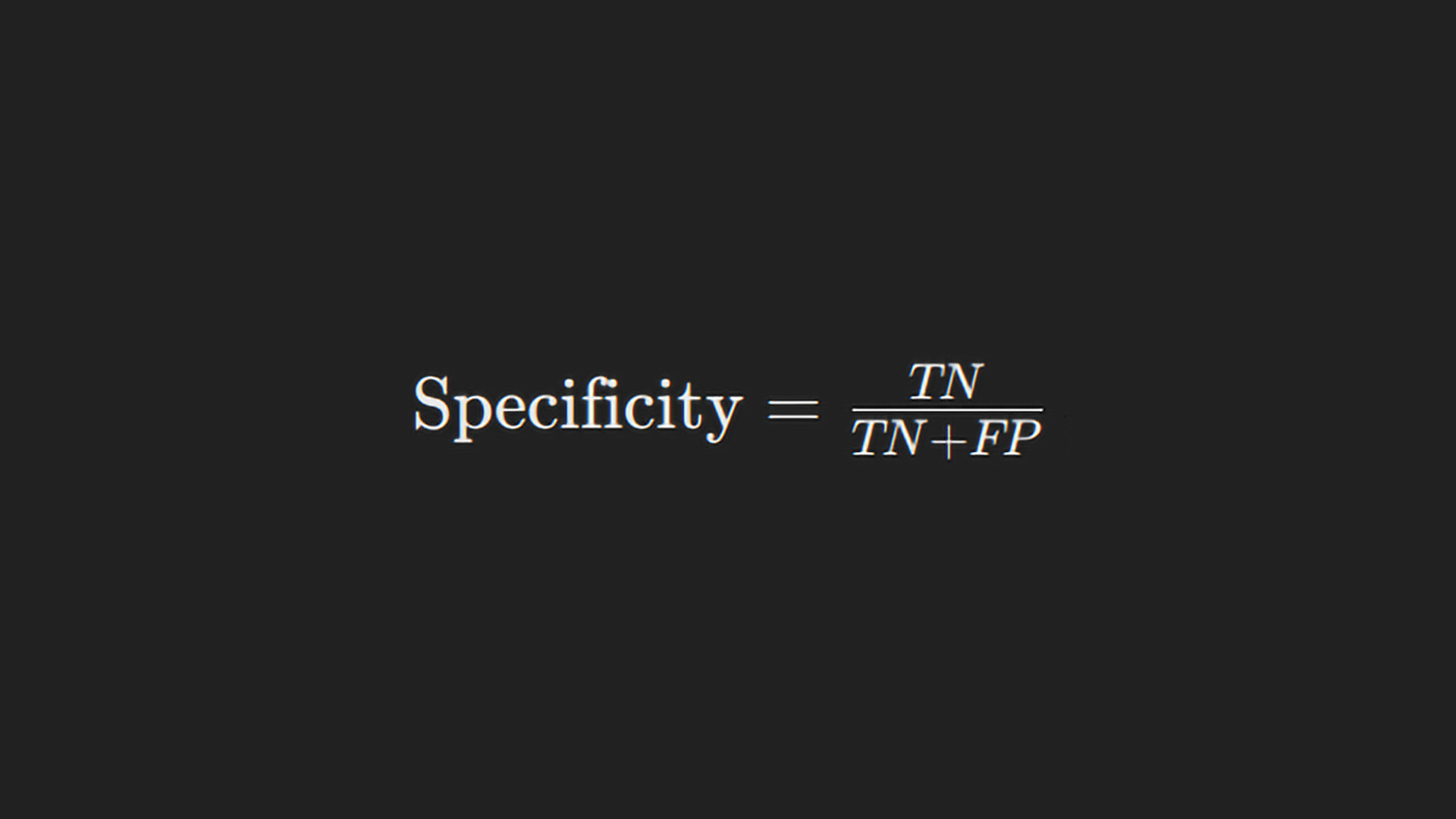
Specificity measures how properly a check can inform a unfavourable. In different phrases, throughout medical screening, specificity exhibits what number of wholesome people who don’t have the illness could also be accurately recognized as externalities.
Sensitivity (or recall in knowledge science) measures the proportion of true constructive predictions out of all constructive circumstances. It solutions the identical query as recall.
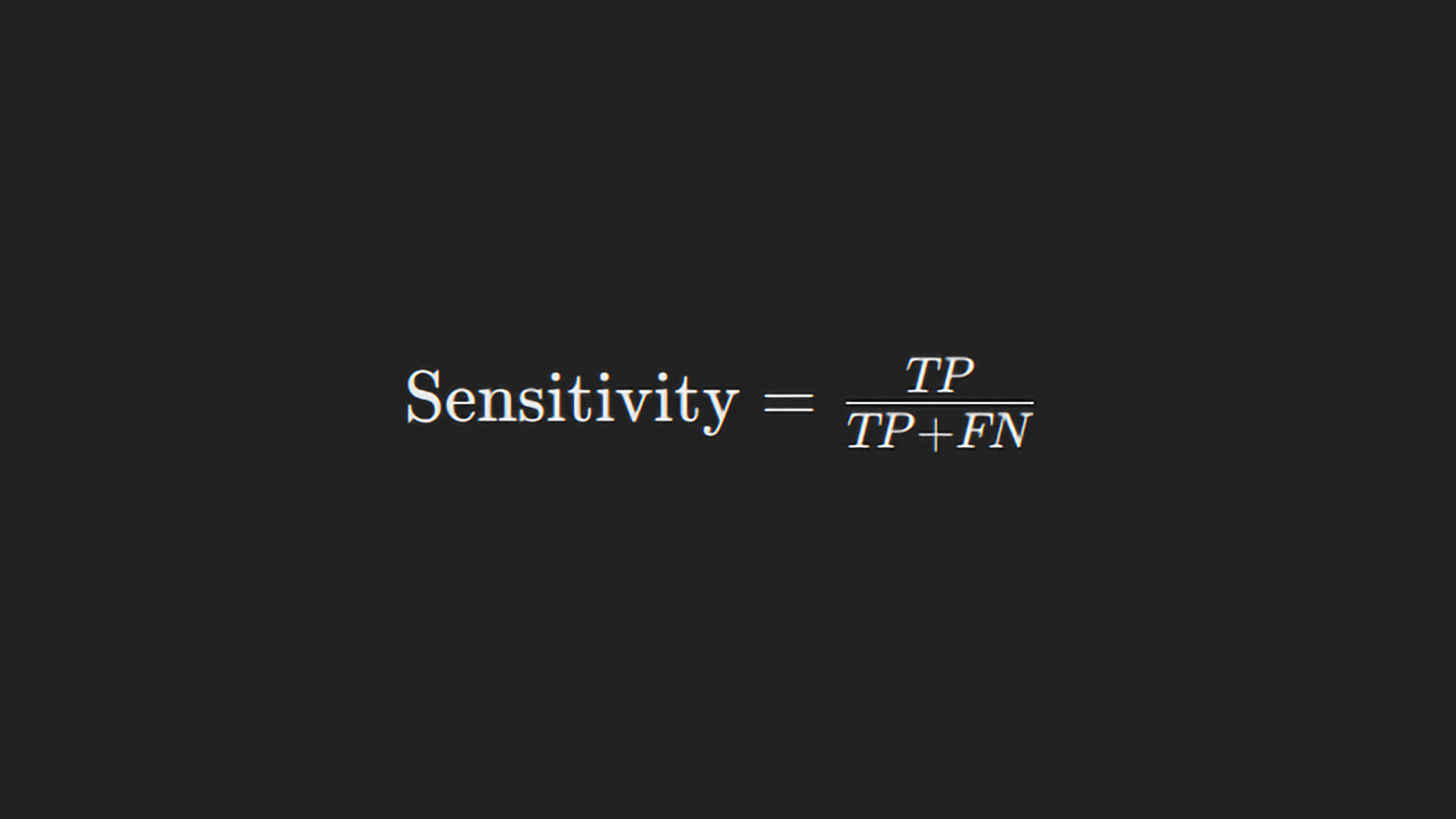
Evaluating Metrics
Precision In comparison with Specificity
Precision and specificity cowl completely different parts of mannequin efficiency. Precision focuses on the accuracy of constructive predictions, asking how lots of the predicted positives are literally constructive. Specificity evaluates the accuracy of unfavourable predictions, indicating how properly the mannequin identifies unfavourable circumstances.
For instance, in a medical check for a uncommon illness, excessive precision implies that most positives recognized even have it, whereas excessive specificity implies that most negatives are accurately categorised as not having it.
Recall vs Sensitivity
The identical metric has two completely different names: recall and sensitivity. Each measures describe what number of true positives the mannequin identifies. Each metrics measure the power to seek out constructive cases, like detecting all sufferers with illness.
Additionally learn: Extracting Medical Data From Medical Textual content With NLP
Sensible Examples
As an example the variations and significance of those metrics, contemplate the next examples:
Instance 1: Low Precision, Excessive Recall, Excessive Specificity
On this situation, if the classifier predicts negatively, the prediction is reliable (excessive specificity), however a constructive prediction is much less dependable (low precision). Nonetheless, the mannequin successfully identifies all constructive circumstances (excessive recall).
One of these classifier may be utilized in preliminary medical screenings the place it’s essential to not miss any constructive circumstances, even when it means having extra false positives.
Instance 2: Excessive Precision, Excessive Recall, Low Specificity
Right here, the classifier predicts every thing as constructive. Whereas it identifies all precise positives (excessive recall) and most predictions are appropriate (excessive precision), it fails to establish negatives (low specificity).
This situation would possibly happen the place lacking a constructive case is extremely undesirable, resembling in essential illness detection, however the place the price of false positives is comparatively low.
Instance 3: Excessive Precision, Low Recall, Excessive Specificity
This classifier is dependable when it predicts a constructive case (excessive precision), however it misses many precise positives (low recall). It accurately identifies most negatives (excessive specificity).
Such a classifier could possibly be used when confidence in constructive predictions is essential, resembling diagnosing a situation requiring extremely invasive or dangerous therapy.
Additionally learn: Utility of Machine Studying in Medical Area!
Selecting the Proper Metric
The proper metric is dependent upon the actual utility and the relative prices of false positives and negatives:
- By way of precision, it’s extra essential to reduce false constructive outcomes when they’re extra essential. As an illustration, in e-mail spam detection, it’s higher to have some spam messages in your inbox than to categorise them as essential spam emails.
- Recall (Sensitivity) issues most if false negatives are essential. For instance, in medical diagnostics, lacking out on a constructive case like a illness might be devastating; therefore, it’s higher to generate some mistaken outcomes that may be eradicated by means of additional checks.
- Specificity assumes significance when the worth of false positives turns into insufferable. As an illustration, in drug testing, false positives needs to be prevented in order to not punish harmless individuals.
Balancing Metrics
One has to strike a stability amongst these ratios. As an illustration, F1 rating is likely one of the metrics that mix each precision and recall to present an general accuracy of a check that balances the trade-off between precision and recall.
F1 rating is extremely beneficial to realize this equilibrium between precision and recall, particularly when imbalanced lessons are concerned.
Conclusion
Understanding and appropriately making use of precision, recall, specificity, and sensitivity are very important for creating and evaluating knowledge science and medication fashions. Every metric supplies distinctive insights into mannequin efficiency, and selecting the best one is dependent upon the particular context and the results of errors. By bridging the hole between these fields, we will enhance communication and collaboration, in the end enhancing the effectiveness of predictive fashions in medical functions.
In abstract, whereas precision and recall are sometimes emphasised in knowledge science and specificity and sensitivity in medication, recognizing their relationships and variations permits for extra nuanced and correct mannequin evaluations. This understanding can considerably affect the event of higher diagnostic instruments and predictive fashions, resulting in improved affected person outcomes and extra environment friendly medical practices.
Be a part of the Licensed AI & ML BlackBelt Plus Program for customized studying tailor-made to your objectives, customized 1:1 mentorship from business consultants, and devoted job placement help. Enroll now and remodel your future!
Regularly Requested Questions
A. Precision measures the accuracy of constructive predictions, whereas recall (sensitivity) assesses the power to establish all precise constructive circumstances.
A. Specificity evaluates the accuracy of unfavourable predictions, indicating how properly a check identifies true negatives, whereas sensitivity (recall) measures the proportion of true positives accurately recognized.
A. Knowledge scientists deal with precision and recall to evaluate mannequin efficiency, whereas medical professionals use specificity and sensitivity to guage diagnostic checks, reflecting their completely different priorities in error administration.
A. Excessive specificity is essential when it is very important precisely establish true negatives, resembling in medical screenings the place false positives can result in pointless nervousness and extra testing.
A. The F1 rating is a metric that balances precision and recall, offering an general measure of a mannequin’s accuracy, particularly helpful when coping with imbalanced lessons.