By: Ankur Gupta & Swagata Ashwani
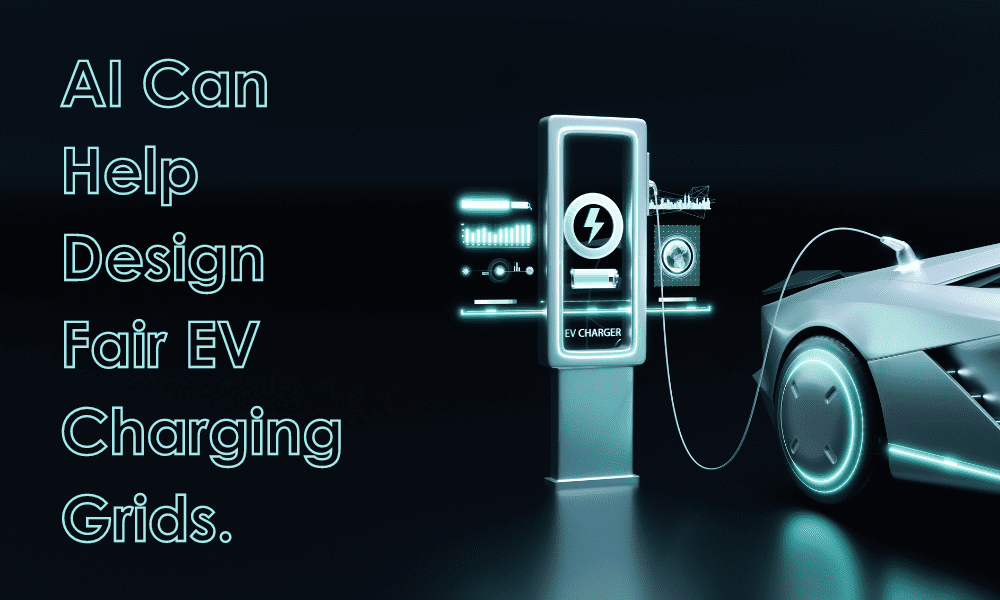
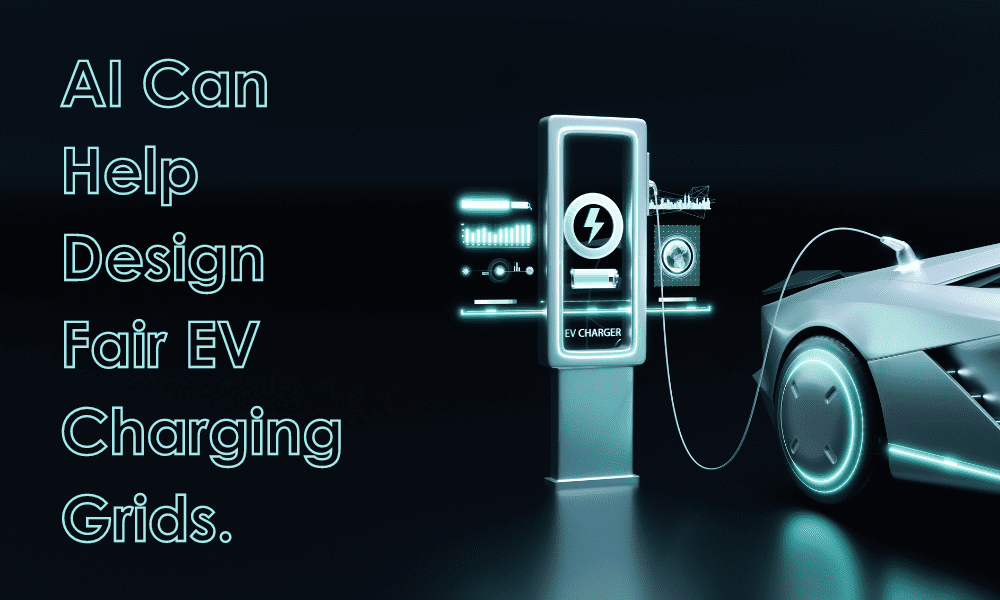
Picture by Editor
Synthetic intelligence holds immense promise for revolutionizing the accessibility and availability of electrical car charging. The demand for EV charging is exploding because the transportation business undergoes an enormous shift in the direction of electrical automobiles. Over 6.5 million EVs had been bought worldwide in 2021, accounting for 9% of passenger automotive gross sales. That quantity ought to exceed 25% by 2030. A latest evaluation estimated that the variety of charging stations required to to fulfill the charging demand would wish to develop 10x by 2040 [1].
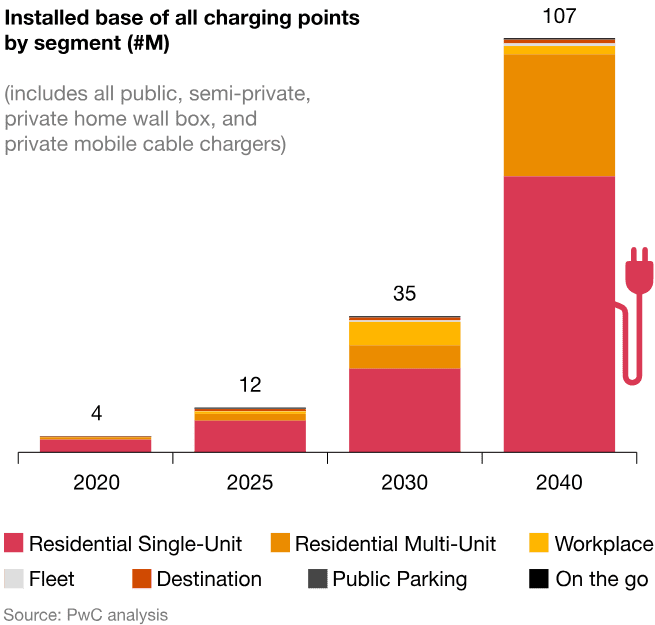
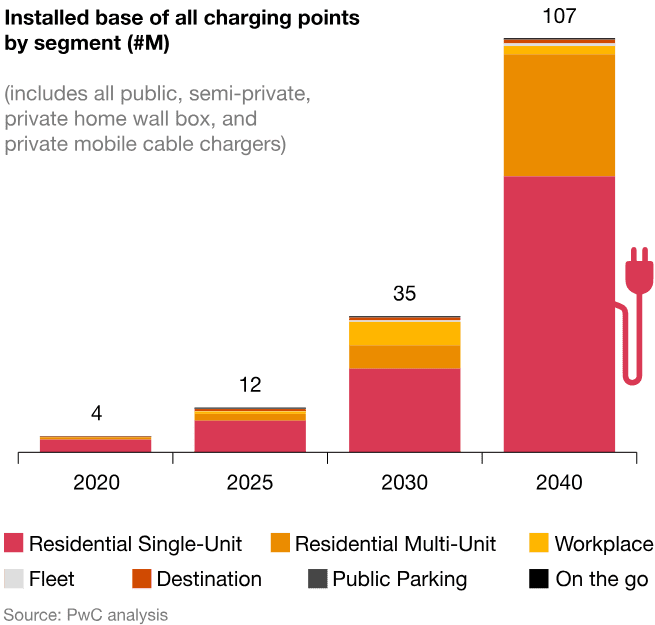
Determine 1: Projected demand for EV charging stations by sort
AI algorithms might help create a better, extra responsive charging infrastructure. Nonetheless, as we welcome the advantages, we should additionally navigate the fast deployment, we additionally want to make sure that it aligns with values comparable to equity, transparency, and accountability.
The datasets that feed into AI fashions would base their suggestions on present EV adoption in these areas, EV demand and anticipated charger utilization. Nonetheless, we have to management for bias primarily based on socio-economic components to make sure that new stations which are positioned on the grid allow honest and equitable entry.
There are additionally myriad scientific research [2,3] that debate how AI and machine studying can be utilized to assist planners resolve the place to find EV chargers and what sort of chargers to put in. Designing an EV charging grid is a posh drawback and varied components are at play together with
charger location, pricing, sort of charging commonplace, charging velocity, vitality grid balancing in addition to predicting the demand. Let’s dive deeper into the important thing elements the place AI might help information us in making a greater resolution.
1. Optimum Charging Station Placement
AI excels in processing huge datasets and extracting significant insights. This functionality turns into significantly helpful when figuring out the optimum areas for charging stations. By analyzing components comparable to visitors patterns, inhabitants density, and geographic information, AI algorithms can strategically place charging stations to maximise accessibility and consumer comfort.
For instance, EV charging stations may be wanted alongside busy commuting routes, close to main highways, or in areas with excessive concentrations of EVs. Excessive-density residential and business areas are prone to have a better demand for EV charging stations. AI can analyze demographic information and inhabitants density maps to pinpoint these areas. For the evaluation, the datasets want to include future tendencies in EV gross sales, inhabitants development and concrete growth.
The Greatest Web site for Charging Stations:
AI algorithms are very good at analyzing large information. They might help to find out the very best areas for EV charging stations. Varied elements are thought of on this evaluation together with:
- Visitors Patterns: AI appears at visitors flows and congestion ranges in order to determine areas with excessive utilization.
- Inhabitants Density: The precedence is given to locations with excessive inhabitants densities thus making certain that there’s most accessibility.
- Geographic Knowledge: This includes analyzing the bodily terrain and constraints of city planning to evaluate their appropriateness.
- Current Charging Station Areas: So as to not saturate any space and preserve a fair unfold.
- Predictive Evaluation for Future Growth: AI makes use of tendencies in electrical car gross sales, demographic shifts and concrete growth to challenge future necessities that information long-term planning.


Determine 2: Warmth map showcasing distribution of EV charging station within the US
2. Demand Prediction
An efficient demand prediction technique is essential for optimizing the position and operation of charging stations and is important for a number of important causes. Firstly, correct demand prediction permits for the strategic placement of charging stations. By forecasting when and the place charging wants might be highest, AI-driven methods can optimize the geographic distribution of charging infrastructure. This ensures that charging stations are conveniently situated in areas with anticipated excessive demand, selling accessibility for a various vary of customers throughout city and rural landscapes.
Secondly, demand prediction contributes to efficient capability planning. By analyzing historic information and incorporating components comparable to differences due to the season, time-of-day patterns, and consumer behaviors, AI might help decide the optimum capability for every charging station. This ensures that the infrastructure is designed to fulfill demand with out inflicting overloads or inefficiencies within the energy grid. Listed under are components that feed into demand prediction.
- EV Charging Transaction Knowledge:
- Particulars about every charging session (time, length, location)
- Power consumed per charging session
- Sort of charging (quick charging, gradual charging)
- Visitors and Mobility Knowledge:
- GPS information from automobiles to know journey patterns
- Visitors move information in several areas and at completely different occasions of the day
- Person Demographics:
- Age, gender, and residential location of EV customers
- Climate:
- Climate situations can have an effect on driving patterns
- Socioeconomic Knowledge:
- Earnings ranges
- City versus rural areas
Predicting demand is essential for consumer satisfaction. Customers profit from a charging infrastructure that aligns with their wants, minimizing wait occasions and offering a seamless expertise. AI’s means to research various datasets, together with consumer conduct and preferences, permits for customized and user-centric demand prediction, enhancing the general satisfaction of EV homeowners
3. Dynamic Charging Pricing Fashions
Conventional fastened pricing fashions could not harness the total potential of a dynamic and responsive charging grid. AI can analyze real-time information, together with vitality demand, grid load, and consumer conduct, to implement dynamic pricing fashions. This not solely optimizes the utilization of the charging infrastructure but in addition encourages customers to cost throughout off-peak hours, selling a extra balanced and sustainable vitality distribution. A analysis examine [4] on dynamic pricing scheme primarily based on the Stackelberg sport for EV charging stations led to the conclusion {that a} effectively crafted pricing scheme can result in discount within the promoting value of charging station whereas rising the station’s revenue; a win-win for each the patron and the supplier.
Elements that feed right into a pricing mannequin:
- Power Demand and Grid Load: AI algorithms can make the most of real-time electrical energy demand and grid load information. Throughout excessive demand, costs may be elevated, and vice versa.
- Person Habits and Patterns: Evaluation of historic charging information, together with frequency, length, and most well-liked occasions for charging, helps predict future conduct and modify costs accordingly.
- Time of Day/Week and Seasonality: Costs can fluctuate primarily based on the time of day, day of the week, or season, contemplating typical utilization patterns throughout these intervals.
- Sort of Charging (Quick vs. Gradual Charging): Totally different charges may be set for various kinds of charging.


Determine 4: Pricing for EV charging stations within the US
Dynamic pricing fashions play a job in affordability and accessibility. By providing decrease costs throughout off-peak hours or when renewable vitality sources are plentiful, AI-driven methods make electrical charging extra economically viable for a various vary of customers. This method aligns with rules of equity, making certain that the advantages of electrical mobility are accessible to people throughout completely different revenue brackets.
The adoption of AI-driven options in electrical car (EV) charging is quickly advancing, providing potential advantages in effectivity, consumer expertise, and grid administration.
Nonetheless, this technological development additionally raises essential issues round algorithmic equity. Guaranteeing that AI methods in EV charging are honest and unbiased is important to selling equitable entry to charging infrastructure.
Numerous and Consultant Knowledge
To mitigate biases, it is essential to make sure that coaching information is various and consultant of the whole consumer base. This includes amassing information from a broad vary of geographic areas, demographic teams, and charging situations. Inside every dataset biases current within the coaching information have to be recognized and rectified. Under are the assorted elements that have to be thought of when selecting the datasets:
- Geographic Range:
- City and Rural Areas: Incorporating information from each city and rural environments ensures that charging grid designs are inclusive and cater to the wants of various communities.
- Totally different Climates: Local weather variations impression charging behaviors and vitality consumption. Datasets reflecting various local weather situations contribute to sturdy AI fashions.
- Demographic Range:
- Socioeconomic Components: Together with information from varied socioeconomic backgrounds helps keep away from biases and ensures that charging infrastructure is accessible to customers throughout completely different revenue ranges.
- Cultural Concerns: Cultural preferences and way of life variations affect charging habits. Numerous datasets encompassing cultural nuances contribute to extra inclusive charging grid designs.
- Automobile Range:
- Varied EV Fashions: Totally different electrical car fashions have distinct charging necessities. Incorporating information from quite a lot of EVs ensures that the charging infrastructure caters to the specs of assorted automobiles.
- Charging Applied sciences: Datasets ought to account for various charging applied sciences, together with quick charging, commonplace charging, and rising applied sciences, to optimize grid designs accordingly.
- Temporal Range:
- Seasonal Variations: Charging behaviors can fluctuate seasonally. Datasets protecting completely different seasons allow AI methods to adapt charging grid designs to altering climate situations.
- Time-of-Day Patterns: Understanding variations in charging demand all through the day aids in optimizing charging infrastructure for various timeframes.
Whereas constructing an AI mannequin for demand prediction- let’s say predicting the place to put the subsequent EV charging station, it’s essential to make sure a various dataset together with all of the above options is curated.
As soon as the options are curated, is it essential to entry the stability of the dataset. An imbalanced dataset can result in skewed and biased outcomes. The graphs present balanced information for a few of the pivoted options comparable to Age and Automobile sort desire.
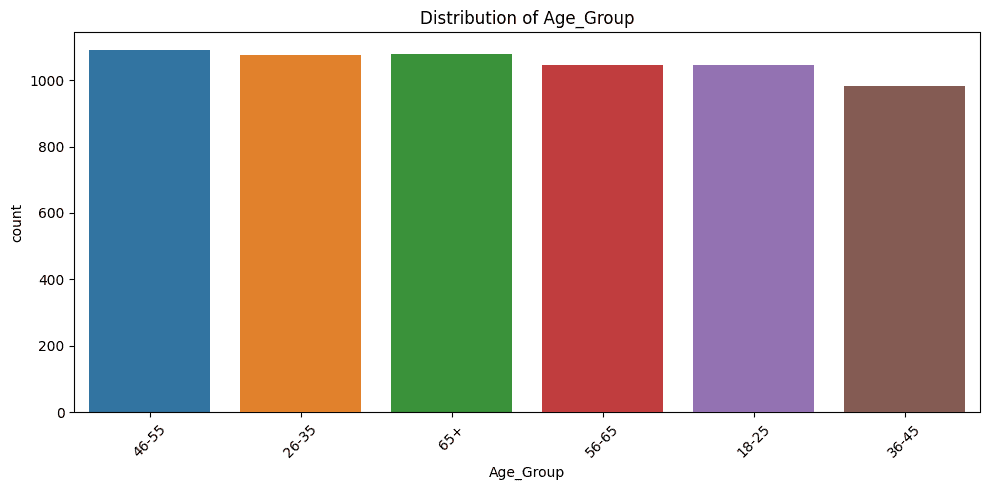
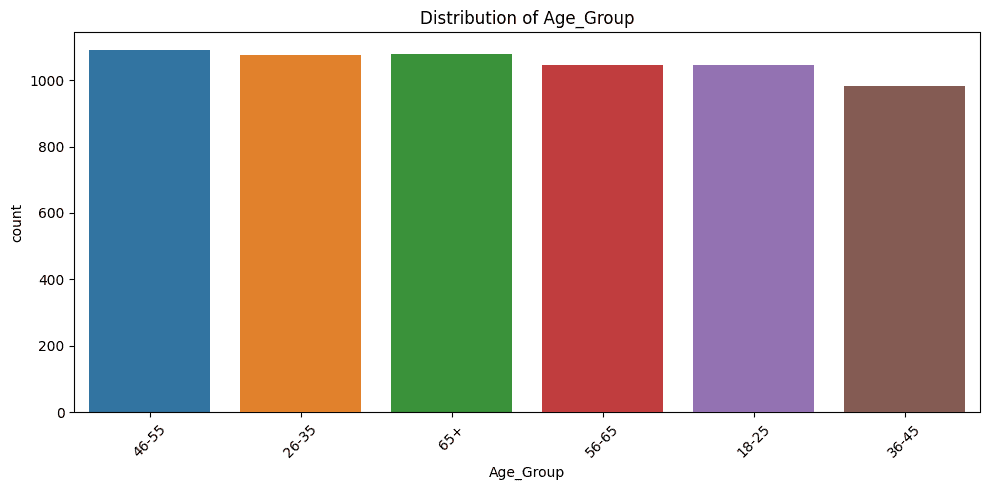
Determine 5: Balanced options for EV charging station placement mannequin by age


Determine 6: Balanced options for EV charging station placement mannequin by car sort
Algorithmic Transparency
Transparency is a cornerstone of addressing bias in AI. Charging algorithms must be designed to be clear, offering customers with insights into how selections are made relating to charging charges, optimum occasions, and different important components. Understanding the algorithm’s decision-making course of fosters belief and permits customers to carry charging suppliers accountable.
LIME (Native Interpretable Mannequin-agnostic Explanations) performs an important position in enhancing the explainability of AI predictions. By creating interpretable fashions that approximate the predictions of advanced machine studying fashions, LIME gives insights into how completely different options affect these predictions. As an example, within the context of EV charging station placement, LIME might help reveal the explanations behind a mannequin’s suggestion to put a charging station- within the under explainer plot- the options that contribute positively to the prediction(putting an EV charging station at location x) is very impacted by the socio-economic standing. Visitors and inhabitants density negatively impression the prediction. That is only a hypothetical dataset and evaluation, and actual life predictions might deeply fluctuate. The aim of this plot is to point out how highly effective LIME may be to clarify how a specific prediction is made- what options carry extra significance vs the others.


Determine 7: Explainable AI for a EV Charging Station prediction utilizing LIME
The EVI-Fairness: Electrical Automobile Infrastructure for Fairness Mannequin developed by NREL [5] is a incredible instrument for measuring the fairness of the nationwide electrical car (EV) charging infrastructure utilizing complete, high-resolution evaluation. It gives a visualization map to permit stakeholders to look at fairness traits of EV charging infrastructure making it simple to examine and perceive the outcomes. For eg. when utilized to the larger Chicago area, the graph under illustrates the disparate charging entry and related EV adoption primarily based on revenue and race.


Determine 8: EVI-Fairness mannequin outcomes for the larger Chicago area
Defending Person Privateness
With the fast rise of Linked Automobiles, there’s an rising quantity of information being streamed from automobiles to the cloud. This not solely contains car metrics comparable to battery capability, remaining vary, consumer settings comparable to local weather management, but in addition driver conduct metrics comparable to price of acceleration/braking, video and audio feeds, anti-braking/lane departure sensor activation. These metrics, if used unfairly, can be utilized to create a behavioral profile for the driving force and in-turn add bias into resolution making.
As AI processes this huge quantity of consumer information to optimize charging grid placement, privateness turns into a paramount concern. Implementing privacy-by-design rules ensures that AI-driven charging infrastructure respects consumer privateness and complies with information safety rules.
Privateness Strategies for Accountable Knowledge Dealing with:
- Anonymization: Anonymization includes the removing or encryption of personally identifiable info from the information stream. By dissociating the information from particular people, it turns into considerably tougher to hint metrics again to a specific driver.
- Aggregation: Aggregation includes combining a number of information factors to kind generalized summaries. As an alternative of processing particular person driver conduct metrics, AI can analyze aggregated patterns throughout a bigger dataset. This not solely safeguards the privateness of particular person drivers but in addition ensures that charging grid selections are primarily based on collective tendencies fairly than particular consumer profiles.
- Differential Privateness: Differential privateness provides noise or randomness to particular person information factors, making it difficult to find out the contribution of a single consumer to the dataset. This system strikes a stability between information utility and privateness safety, enabling AI to generate correct charging grid optimizations with out compromising the person privateness of drivers.
- Homomorphic Encryption: Homomorphic encryption permits computations on encrypted information with out decrypting it. This system permits AI to research encrypted driver conduct metrics, making certain that the privateness of particular person customers is maintained all through the optimization course of. It is a highly effective instrument for placing a stability between data-driven insights and privateness safety.
As the worldwide adoption of electrical automobiles (EVs) good points momentum, charging networks infused with AI face each promising alternatives and vital tasks. Their mission includes offering drivers with comfort and reliability whereas making certain the resilience of native grids, all whereas prioritizing fairness and accountability. Though the challenges are intricate, the potential future advantages are immense, starting from cleaner air and local weather change mitigation to reaching vitality independence and fostering the event of next-generation expertise.
The pivotal position of AI and machine studying in bringing this imaginative and prescient to fruition can’t be overstated. These applied sciences maintain the promise of orchestrating serialized, customized charging on an enormous scale, catering to tens of millions of customers. Nonetheless, to safe public belief, the algorithms driving these methods should middle on rules of equity and transparency, all whereas enhancing accessibility and reliability.
[1] US electrical car charging market development: PwC
[2] The position of synthetic intelligence within the mass adoption of electrical automobiles
[3] Knowledge-driven sensible charging for heterogeneous electrical car fleets – ScienceDirect
Swagata Ashwani is a seasoned information scientist with a wealthy background in analytics and large information. At present serving because the Principal Knowledge Scientist at Boomi, Swagata performs an important position in harnessing the facility of information to drive innovation and effectivity. In her position, she performs an important position in main generative AI initiatives for the corporate. She can be Chapter Lead at SF Ladies in Knowledge, the place she fosters constructing a wealthy neighborhood for girls to have a good time ladies in assorted information roles.
Ankur Gupta is an engineering chief with a decade of expertise spanning sustainability, transportation, telecommunication and infrastructure domains; presently holds the place of Engineering Supervisor at Uber. On this position, he performs a pivotal position in driving the development of Uber’s Automobiles Platform, main the cost in the direction of a zero-emissions future by way of the combination of cutting-edge electrical and related automobiles.