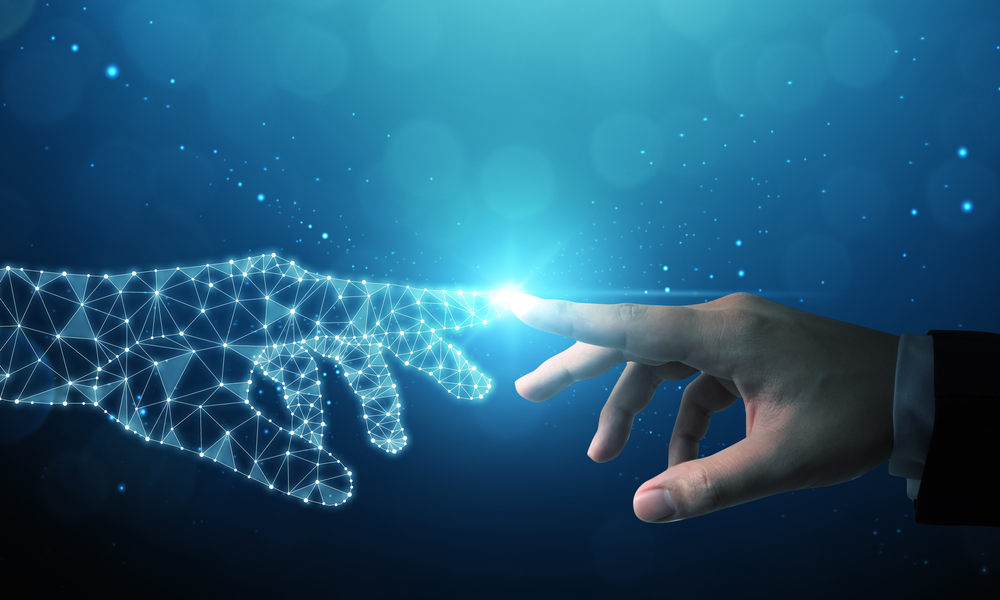
(Monster Ztudio/Shutterstock)
Synthetic Intelligence (AI) is present process a profound transformation, presenting immense alternatives for companies of all sizes. Generative AI has changed conventional ML and AI as the recent subject in boardrooms. Nevertheless, a latest Boston Consulting Group (BCG) examine exhibits that greater than half of the executives surveyed need assistance understanding GenAI. They’re actively discouraging its use, whereas an additional 37% point out they’re in a state of experimentation however haven’t any insurance policies or controls in place. Within the following article, I’ll delve into the widespread accessibility of AI, analyze the related obstacles and benefits, and find out about methods for organizations to adapt to this ever-evolving discipline.
Corporations ought to align governance and accountable AI practices with tangible enterprise outcomes and danger administration. Demonstrating how adherence to those tips can profit the group ethically and concerning bottom-line outcomes helps garner stakeholder assist and dedication in any respect ranges.
Differentiating AI: Conventional vs. Generative AI
Distinguishing between conventional AI and generative AI is essential for greedy the complete scope of AI democratization. Conventional AI, which has existed for many years, supplied a method to investigate huge quantities of information to outline a rating or a sample based mostly on the learnings from the information. However the solutions are all the time predictable – i.e., if the identical query is requested ten occasions, the reply would stay the identical. Creating the prediction or rating usually calls for a specialised workforce of information scientists and specialists to construct and deploy fashions, making this much less accessible to a broader viewers inside organizations.
Generative AI, then again, represents a paradigm shift. It encompasses applied sciences like giant language fashions that may create content material in a human-like vogue based mostly on the large quantities of information used to coach these fashions. Along with the system with the ability to create new content material (textual content, photos, video, audio, and so forth.), it should always study and evolve to the purpose that responses are not predictable or deterministic however will hold altering. This shift democratizes AI by making it accessible to a broader vary of customers, no matter their specialised ability units.
Balancing the Challenges and Dangers of Fast AI Adoption
Generative AI introduces distinctive challenges, primarily when counting on prepackaged options. The idea of explainability in AI presents a big problem, notably in conventional AI programs the place outcomes are sometimes offered as easy likelihood scores like “0.81” or “mortgage denied.” Deciphering the reasoning behind such scores usually requires specialised data, elevating questions on equity, potential biases stemming from profiling, and different elements influencing the end result.
When discussing explainability inside the realm of GenAI, it’s essential to look at the sources behind the reasons supplied, notably within the case of open LLMs resembling OpenAI or Llama. These fashions are skilled on huge quantities of web information and GitHub repositories, elevating issues in regards to the origin and accuracy of responses and potential authorized dangers associated to copyright infringement. Furthermore, fine-tuning embeddings usually feed into vector databases, enriching them with qualitative data. The query of information provenance stays pertinent. Nevertheless, if somebody have been to enter their assist tickets into the system, they’d have a clearer understanding of the information’s origins.
Whereas the democratization of GenAI presents immense worth, it additionally introduces particular challenges and dangers. The fast adoption of GenAI can result in issues associated to information breaches, safety vulnerabilities, and governance points. Organizations should strike a fragile stability between capitalizing on the advantages of GenAI and guaranteeing information privateness, safety, and regulatory compliance.
It’s crucial to obviously perceive the dangers, sensible options, and finest practices for implementing accountable GenAI. When workers perceive the potential dangers and the methods to navigate stated dangers, they’re extra prone to embrace accountable GenAI practices and are higher positioned to navigate challenges successfully. Taking a balanced strategy fosters a tradition of accountable AI adoption.
Accountable AI: Bridging the Hole Between Intent and Motion
Organizations are more and more establishing accountable GenAI charters and evaluation processes to deal with the challenges of GenAI adoption. These charters information moral GenAI use and description the group’s dedication to accountable GenAI practices. Nevertheless, the crucial problem is bridging the hole between intent and motion when implementing these charters. Organizations should transfer past ideas to concrete actions that guarantee GenAI is used responsibly all through its lifecycle.
To maximise AI’s advantages, organizations ought to encourage completely different groups to experiment and develop their GenAI apps and use circumstances whereas offering prescriptive steerage on the required controls to stick to and which instruments to make use of. This strategy ensures flexibility and adaptableness inside the group, permitting groups to tailor options to their particular wants and targets.
Constructing a Framework That Opens Doorways to Transparency
AI is a dynamic discipline characterised by fixed innovation and evolution. In consequence, frameworks for accountable AI should be agile and able to incorporating new learnings and updates. Organizations ought to undertake a forward-looking strategy to accountable AI, acknowledging that the panorama will proceed to evolve. As transparency turns into a central theme in AI governance, rising rules pushed by organizations just like the White Home could compel AI suppliers to reveal extra details about their AI programs, information sources, and decision-making processes.
Efficient monitoring and auditing of AI programs are important to accountable AI practices. Organizations ought to set up checkpoints and requirements to make sure compliance with accountable AI ideas. Common inspections, performed at intervals resembling month-to-month or quarterly, assist preserve the integrity of AI programs and guarantee they align with moral tips.
Privateness vs. AI: Evolving Issues
Privateness issues aren’t new and have existed for a while now. Nevertheless, the worry and understanding of AI’s energy have grown lately, contributing to its reputation throughout industries. AI is now receiving elevated consideration from regulators at each the federal and state ranges. Rising issues about AI’s impression on society and people are resulting in heightened scrutiny and requires regulation.
Enterprises ought to embrace privateness and safety as enablers somewhat than viewing them as obstacles to AI adoption. Groups ought to actively search methods to construct belief and privateness into their AI options whereas concurrently reaching their enterprise objectives. Putting the appropriate stability between privateness and AI innovation is important.
Democratization of AI: Accessibility and Productiveness
Generative AI’s democratization is a game-changer. It empowers organizations to create productivity-enhancing options with out requiring intensive information science groups. For example, gross sales groups can now harness the facility of AI instruments like chatbots and proposal turbines to streamline their operations and processes. This newfound accessibility empowers groups to be extra environment friendly and artistic of their duties, finally driving higher outcomes.
Shifting Towards Federal-Degree Regulation and Authorities Intervention
Generative AI regulatory frameworks will transfer past the state stage in direction of federal and country-level requirements. Numerous working teams and organizations are actively discussing and growing requirements for AI programs. Federal-level regulation may present a unified framework for accountable AI practices, streamlining governance efforts.
Given the broad implications of AI decision-making, there’s a rising expectation of presidency intervention to make sure accountable and clear AI practices. Governments could assume a extra energetic position in shaping AI governance to safeguard the pursuits of society as an entire.
In conclusion, the democratization of AI signifies a profound shift within the technological panorama. Organizations can harness AI’s potential for enhanced productiveness and innovation whereas adhering to accountable AI practices that defend privateness, guarantee safety, and uphold moral ideas. Startups, specifically, are poised to play a significant position in shaping the accountable AI panorama. Because the AI discipline evolves, accountable governance, transparency, and a dedication to moral AI use will guarantee a brighter and extra equitable future for all.
Concerning the creator: Balaji Ganesan is CEO and co-founder of Privacera. Earlier than Privacera, Balaji and Privacera co-founder Don Bosco Durai additionally based XA Safe. XA Safe was acquired by Hortonworks, who contributed the product to the Apache Software program Basis and rebranded as Apache Ranger. Apache Ranger is now deployed in hundreds of corporations all over the world, managing petabytes of information in Hadoop environments. Privacera’s product is constructed on the inspiration of Apache Ranger and supplies a single pane of glass for securing delicate information throughout on-prem and a number of cloud companies resembling AWS, Azure, Databricks, GCP, Snowflake, and Starburst and extra.
Associated Gadgets:
GenAI Doesn’t Want Greater LLMs. It Wants Higher Knowledge
Prime 10 Challenges to GenAI Success
Privacera Report Reveals That 96% of Companies are Pursuing Generative AI for Aggressive Edge