Introduction
Floods disproportionately impression creating international locations with sparse streamflow gauge networks, highlighting the necessity for correct early warnings. The acceleration of flood-related disasters attributable to local weather change underscores the urgency for efficient early warning programs, particularly in low- and middle-income international locations the place 90% of susceptible populations reside. In keeping with the World Financial institution, upgrading flood early warning programs in creating nations to developed requirements might save a median of 23,000 lives yearly. Nonetheless, challenges persist, together with the need for particular person watershed calibration and restricted forecasting in susceptible areas. On this article, we’ll perceive the analysis paper by Google that explores utilizing synthetic intelligence (AI) to foretell riverine flooding as much as 5 days upfront, detailing its potential implications for over 80 international locations, significantly in data-scarce and susceptible areas.
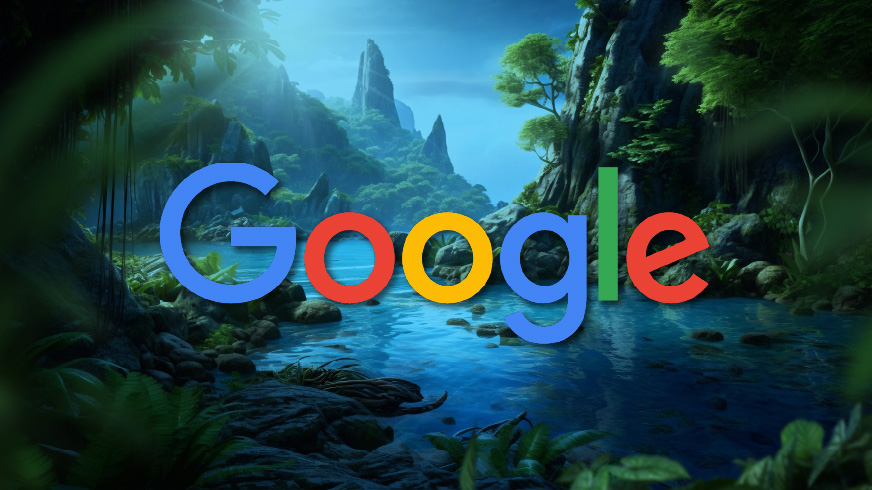
The Devastating Impression of Floods
Floods are the commonest kind of pure catastrophe, and the speed of flood-related disasters has greater than doubled since 2000. This enhance is attributed to the accelerating hydrological cycle attributable to anthropogenic local weather change. Flood impacts are significantly extreme in creating international locations, the place populations are extremely susceptible to flood dangers. The devastating penalties of floods underscore the pressing want for correct and well timed flood warnings to mitigate the impression on human lives and property.
The Present State of Flood Forecasting
The present state of flood forecasting faces challenges, significantly in ungauged basins the place hydrological prediction fashions want extra dependable information for calibration. This limitation hinders flood forecasts’ accuracy and lead time, particularly in areas susceptible to flooding’s human impacts. The dearth of dense streamflow gauge networks in creating international locations additional exacerbates the inaccuracy of flood warnings, highlighting the important want for improved world entry to dependable flood forecasts.
A Ray of Hope: Google AI to the Rescue
Google Synthetic Intelligence (AI) presents a promising answer to the challenges of worldwide flood forecasting. By leveraging AI and open datasets, there may be potential to considerably enhance the precision, recall, and lead time of short-term forecasts of maximum riverine occasions. The event of an operational system that produces publicly accessible forecasts in real-time in over 80 international locations demonstrates the potential of AI to offer early and correct flood warnings in ungauged basins. This marks a major development in enhancing world entry to dependable flood forecasts and early warning programs.
Google Analysis Paper: AI Revolutionizes Flood Forecasting
The Google analysis paper presents a major development in flood forecasting utilizing synthetic intelligence (AI) educated on open and public datasets. The research evaluates the potential of AI to revolutionize world entry to forecasts of maximum occasions in worldwide rivers. By leveraging AI, an operational system has been developed to supply short-term (7-day) flood forecasts in over 80 international locations, offering real-time forecasts with out obstacles to entry, corresponding to financial costs or web site registration.
Utilizing AI for World Flood Forecasts
The Google analysis paper delves into the usage of AI for world flood forecasts, highlighting the event of an AI streamflow forecasting mannequin that extends earlier work on hydrological nowcast fashions. The mannequin makes use of lengthy short-term reminiscence (LSTM) networks to foretell day by day streamflow by a 7-day forecast horizon. Notably, the AI mannequin doesn’t use streamflow information as inputs, addressing the problem of real-time information availability, particularly in ungauged areas. The mannequin structure incorporates an encoder-decoder mannequin with separate LSTM items for historic and forecast meteorological enter information.
From Open Information to Actual-Time Forecasts
The operational system developed primarily based on the AI mannequin supplies real-time flood forecasts in over 80 international locations, marking a major milestone in enhancing world entry to dependable flood warnings. The system’s potential to supply short-term forecasts with out obstacles to entry, as demonstrated by the provision of forecasts in real-time
and freed from cost, underscores the potential of AI to reinforce early warning programs for flood occasions.
Past State-of-the-Artwork
The AI mannequin’s efficiency surpasses the present state-of-the-art world modeling system, the Copernicus Emergency Administration Service World Flood Consciousness System (GloFAS). The research experiences that AI-based forecasting achieves reliability in predicting excessive riverine occasions in ungauged watersheds at a lead time of as much as 5 days, similar to or higher than the reliability of nowcasts from GloFAS. Moreover, the AI mannequin’s accuracy over five-year return interval occasions is just like or higher than present accuracies over one-year return interval occasions, indicating its potential to offer early and correct flood warnings over bigger and extra impactful occasions in ungauged basins.
Below the Hood: The AI Mannequin
Constructing the Brains
The AI streamflow forecasting mannequin extends earlier work on hydrological nowcast fashions utilizing LSTM networks to simulate sequences of streamflow information from meteorological enter information. The mannequin makes use of an encoder–decoder structure with one LSTM operating over a historic sequence of meteorological enter information (the encoder LSTM) and one other LSTM operating over the 7-day forecast horizon with inputs from meteorological forecasts (the decoder LSTM). The mannequin doesn’t use streamflow information as inputs because of the unavailability of real-time information in ungauged areas, and the benchmark (GloFAS) doesn’t use autoregressive inputs. The dataset consists of mannequin inputs and streamflow targets for 152,259 years from 5,680 watersheds, with a complete measurement of 60 GB saved to disk.
The Information Timeline
The determine reveals the accessible information intervals from every supply used for coaching and prediction with the AI mannequin. Throughout coaching, lacking information was imputed both by utilizing an identical variable from one other information supply or by imputing with a imply worth and including a binary flag to point an imputed worth. The mannequin makes use of a hindcast sequence size of 12 months, with a hidden measurement of 256 cell states for each the encoder and decoder LSTMs.
How Effectively Does the AI Mannequin Predict?
The efficiency of the AI mannequin was assessed utilizing cross-validation experiments, with information from 5,680 gauges break up in time and area to make sure out-of-sample predictions. The mannequin predicts parameters of a single uneven Laplacian distribution over area-normalized streamflow discharge at every time step and forecast lead time. The mannequin was educated on 50,000 minibatches with a batch measurement of 256 and standardized inputs by subtracting the imply and dividing by the usual deviation of training-period information.
Placing the Mannequin to the Check
The cross-validation experiments included splits throughout continents, local weather zones, and teams of hydrologically separated watersheds. The AI mannequin was evaluated out-of-sample in each location and time, and the outcomes had been reported over a hydrograph that resulted from averaging the expected hydrographs from an ensemble of three individually educated encoder–decoder LSTMs.
Evaluating the Mannequin with Hydrograph Metrics
Hydrograph metrics for the AI mannequin and GloFAS total analysis gauges had been assessed, with scores reducing with rising lead time. The outcomes had been calculated for the time interval 2014-2021, and the metrics had been listed in Prolonged Information Desk 1. Moreover, hydrograph metrics for the AI mannequin and GloFAS over the 1,144 gauges the place GloFAS is calibrated had been evaluated, with scores reducing with rising lead time.
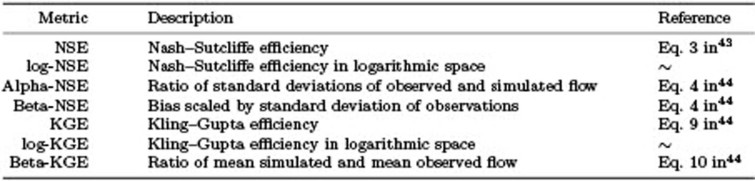
What Makes the AI Tick?
Function significance rankings from reliability classifiers had been used to point which geophysical attributes decide excessive versus low reliability within the AI mannequin. Essentially the most important options of the AI mannequin included drainage space, imply annual potential evapotranspiration (PET), imply annual precise evapotranspiration (AET), and elevation. These attributes had been correlated with reliability scores, indicating a excessive diploma of nonlinearity and parameter interplay within the mannequin.
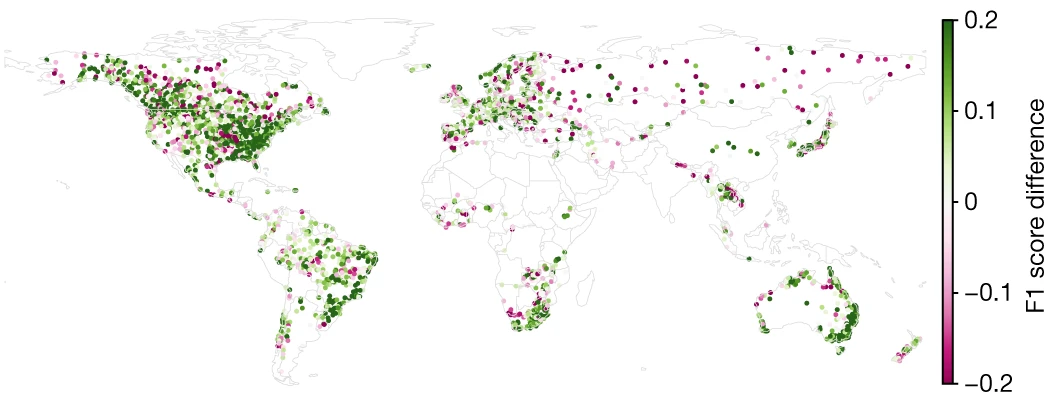
Conclusion
Whereas hydrological modeling has matured, many flood-prone areas lack dependable forecasting and early warning programs. The Google analysis paper demonstrates how leveraging AI and open information can considerably enhance short-term forecasts’ precision, recall, and lead time for excessive riverine occasions. AI-based forecasting provides a promising answer by extending the reliability of present world nowcasts to a 5-day lead time and enhancing forecast abilities in Africa to ranges similar to Europe.
Furthermore, offering these forecasts publicly in real-time with out entry obstacles allows well timed dissemination of flood warnings. Regardless of this progress, there may be room for additional enchancment by rising entry to hydrological information to coach correct fashions and real-time updates by open-source initiatives like Caravan. Enhancing world flood predictions and early alerts is important for safeguarding thousands and thousands worldwide from the devastating impacts of floods on lives and property. Combining AI, open information, and collaborative efforts paves the best way towards this very important purpose.